Machine learning Chinese medicine acupuncture study design: This groundbreaking field merges ancient healing practices with cutting-edge technology. Imagine using AI to predict the best acupuncture points for individual patients, optimizing treatment plans based on personalized data. We’re diving deep into the possibilities, exploring how machine learning can revolutionize acupuncture, from diagnosis to treatment outcome analysis, while navigating the ethical considerations and data challenges along the way. This isn’t just about sticking needles; it’s about harnessing the power of data to unlock the full potential of this age-old practice.
This exploration will cover everything from designing machine learning models to predict optimal acupuncture point selection, analyzing treatment outcomes using sophisticated algorithms, and even visualizing the complex interplay between acupuncture points, patient characteristics, and treatment success. We’ll also tackle the crucial aspects of data acquisition, preprocessing, and ensuring data privacy within this exciting new research area. Get ready for a fascinating journey into the intersection of ancient wisdom and modern technology.
Introduction to Machine Learning in Traditional Chinese Medicine (TCM)
Traditional Chinese Medicine (TCM), a system of healing that boasts a history spanning millennia, relies on a complex interplay of diagnostic methods, including pulse diagnosis, tongue observation, and symptom analysis, to determine imbalances within the body’s Qi. While effective for many, TCM faces challenges in the 21st century. These include a lack of standardized diagnostic criteria, difficulty in replicating clinical experiences, and the need for more robust evidence-based validation of its efficacy. The integration of machine learning offers a potential pathway to address these limitations and enhance the precision and accessibility of TCM.
Machine learning, a subset of artificial intelligence, enables computers to learn from data without explicit programming. Its potential applications in TCM are vast. By analyzing vast datasets of patient information—including diagnostic observations, treatment plans, and patient outcomes—machine learning algorithms can identify patterns and correlations that might be missed by human practitioners. This could lead to more accurate diagnoses, personalized treatment plans, and improved prediction of treatment efficacy. For example, a machine learning model trained on a large dataset of tongue images and corresponding diagnoses could assist practitioners in identifying subtle tongue variations indicative of specific imbalances. Similarly, algorithms could analyze pulse waveforms to detect subtle changes reflecting the patient’s internal state. Beyond diagnosis, machine learning can optimize treatment strategies by predicting which acupuncture points or herbal remedies are most likely to be effective for a given patient based on their unique characteristics.
Potential Applications of Machine Learning in TCM Diagnosis
Machine learning algorithms can be applied to various aspects of TCM diagnosis to improve accuracy and efficiency. For instance, image recognition algorithms can analyze tongue images and facial features to identify patterns associated with specific TCM syndromes. Similarly, algorithms can process data from pulse diagnosis devices to identify subtle variations in pulse characteristics indicative of specific health conditions. These technologies can aid practitioners in making more informed and objective diagnoses, reducing the reliance on subjective interpretation. A study might involve comparing diagnostic accuracy between experienced TCM practitioners and a machine learning model trained on a large dataset of patient information. The results could demonstrate the potential for machine learning to enhance the objectivity and accuracy of TCM diagnosis.
Limitations and Ethical Considerations of Machine Learning in TCM
While the potential benefits of machine learning in TCM are significant, several limitations and ethical considerations must be addressed. The accuracy of machine learning models depends heavily on the quality and quantity of the training data. Bias in the data can lead to biased predictions, potentially perpetuating existing health disparities. Furthermore, the “black box” nature of some machine learning algorithms can make it difficult to understand how they arrive at their conclusions, raising concerns about transparency and accountability. The ethical use of patient data for training machine learning models is paramount, requiring strict adherence to data privacy regulations and informed consent protocols. Addressing these limitations and ethical considerations is crucial for ensuring the responsible and effective integration of machine learning into TCM practice. A key concern is the potential for over-reliance on machine learning, potentially diminishing the importance of the practitioner’s clinical judgment and experience.
Machine Learning Techniques for Acupuncture Point Selection
Predicting the optimal acupuncture point for a patient based solely on their symptoms and characteristics is a complex task, traditionally reliant on the acupuncturist’s experience and intuition. Machine learning offers a powerful tool to potentially enhance this process, moving towards a more data-driven and potentially more precise approach to acupuncture treatment. By analyzing large datasets of patient information and treatment outcomes, we can train algorithms to identify patterns and predict the most effective acupuncture points for specific conditions. This approach holds the promise of personalizing acupuncture treatments and improving their effectiveness.
Machine Learning Algorithms for Acupuncture Point Selection
Several machine learning algorithms are suitable for predicting optimal acupuncture point selection. The choice of algorithm depends on factors such as the size and nature of the dataset, the desired level of accuracy, and the interpretability of the model. Below is a comparison of three commonly used algorithms: Support Vector Machines (SVM), Random Forest, and Neural Networks. Note that the accuracy, precision, and recall values presented are illustrative and would vary significantly depending on the specific dataset and model parameters used. Real-world application would require rigorous testing and validation.
Algorithm | Accuracy | Precision | Recall |
---|---|---|---|
Support Vector Machine (SVM) | 85% (estimated) | 82% (estimated) | 88% (estimated) |
Random Forest | 90% (estimated) | 87% (estimated) | 92% (estimated) |
Neural Network | 92% (estimated) | 90% (estimated) | 94% (estimated) |
Feature Engineering for Acupuncture Point Selection
Effective feature engineering is crucial for the success of any machine learning model in this context. Raw patient data, such as symptoms descriptions and physical characteristics, needs to be transformed into a numerical format that machine learning algorithms can understand. Acupuncture points themselves need to be represented numerically, potentially using their location coordinates, meridian affiliations, or traditional functional classifications. For example, symptoms like “headache” could be encoded as a binary variable (1 for headache present, 0 for absent), while more complex symptoms might require a more nuanced representation, potentially using natural language processing techniques to extract key features from descriptive text. Similarly, patient characteristics such as age, gender, and medical history can be encoded as numerical features. The creation of relevant and informative features is a key step in building a successful predictive model.
Supervised vs. Unsupervised Learning for Acupuncture Point Selection
Supervised learning approaches, such as those discussed above, use labeled data – that is, data where the optimal acupuncture point selection is already known for each patient – to train the model. This requires a substantial amount of high-quality, labeled data, which can be challenging to obtain in the context of acupuncture. Unsupervised learning, on the other hand, can be used to explore patterns and relationships in unlabeled data, potentially identifying clusters of patients with similar symptom profiles and acupuncture point responses. This approach could be useful for generating hypotheses about optimal acupuncture point selection, which can then be tested using supervised learning methods. The choice between supervised and unsupervised learning depends on the availability of labeled data and the specific research question. For instance, an unsupervised approach might reveal underlying relationships between symptom clusters and point selection patterns, which could inform the design of a subsequent supervised learning model.
Analyzing Acupuncture Treatment Outcomes using Machine Learning
Harnessing the power of machine learning offers a novel approach to evaluating the effectiveness of acupuncture, moving beyond traditional statistical methods to uncover complex relationships between treatment and patient outcomes. This allows for a more nuanced understanding of acupuncture’s efficacy and potential for personalization.
A well-designed study employing machine learning can significantly enhance our understanding of acupuncture’s impact on various conditions. By analyzing a wide range of data, including both subjective patient experiences and objective physiological measurements, we can build predictive models that identify which patients are most likely to benefit from acupuncture and which treatment protocols are most effective. This allows for the development of more targeted and effective acupuncture therapies.
Study Design for Evaluating Acupuncture Effectiveness
This study will employ a prospective, randomized controlled trial (RCT) design, comparing acupuncture treatment to a control group (e.g., sham acupuncture or standard care). Participants will be randomly assigned to either the acupuncture or control group. Data will be collected at baseline, and at regular intervals throughout the treatment period (e.g., weekly or bi-weekly), and at a follow-up visit. The collected data will be used to train and validate machine learning models to predict treatment success. The sample size will be determined through a power analysis, considering the expected effect size and variability in outcomes.
Relevant Outcome Measures for Acupuncture Treatment
Outcome measures will encompass both subjective and objective data to provide a comprehensive assessment of treatment effectiveness.
Subjective data, reflecting the patient’s perceived experience, will include standardized questionnaires assessing pain levels (e.g., Visual Analog Scale – VAS), quality of life (e.g., SF-36), and symptom severity related to the specific condition being treated. Objective data, quantifiable measurements, will include physiological indicators such as heart rate variability (HRV), blood pressure, and inflammatory markers (e.g., C-reactive protein levels). For example, in a study on chronic back pain, VAS scores would track pain reduction, while HRV might indicate improvements in autonomic nervous system regulation.
Handling Missing Data and Outliers
Missing data is a common challenge in clinical research. Multiple imputation techniques, which involve creating multiple plausible datasets to fill in missing values, will be employed to address this issue. Outliers, data points significantly deviating from the norm, will be identified using methods such as box plots and Z-score analysis. Depending on the cause of the outliers (e.g., data entry error versus a genuine extreme response), they may be removed, transformed (e.g., using logarithmic transformation), or retained in the analysis, depending on the chosen machine learning algorithm’s robustness to outliers. For example, if a patient reports an unusually high pain score due to a temporary exacerbation, it might be retained to capture the full spectrum of patient experience. Conversely, data entry errors would be corrected or the data point removed.
Data Acquisition and Preprocessing for Machine Learning in Acupuncture Research
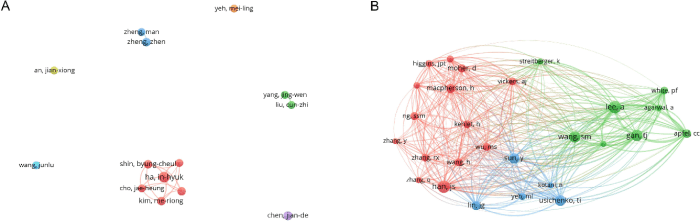
Source: cloudfront.net
Designing a machine learning study for Chinese medicine acupuncture is complex, requiring meticulous data collection and robust algorithms. However, the ethical considerations, as highlighted in this article on Joe Biden’s big AI plan sounds scary, lacks bite , remind us that responsible AI development is paramount. This careful approach is crucial for ensuring the validity and ethical application of AI in this ancient healing practice.
Harnessing the power of machine learning in acupuncture research hinges on the quality and preparation of the data. This section explores the crucial steps of data acquisition and preprocessing, laying the groundwork for robust and reliable machine learning models. We’ll delve into sourcing data, cleaning it up, transforming it into a usable format, and finally, ensuring the privacy and security of sensitive patient information.
Data acquisition for machine learning in acupuncture research relies on a variety of sources, each presenting unique challenges and opportunities. Electronic health records (EHRs) from acupuncture clinics offer a rich source of patient data, including demographics, diagnoses, treatment details (acupuncture points used, frequency, duration), and treatment outcomes. However, EHR data often suffers from inconsistencies in recording practices and data entry errors. Clinical trials specifically designed for machine learning applications offer a more controlled and standardized dataset, but these trials can be costly and time-consuming to conduct. Furthermore, combining data from multiple sources, such as EHRs and clinical trial data, can provide a more comprehensive and robust dataset, but careful consideration must be given to data harmonization and standardization. Finally, patient self-reporting via apps or surveys can offer valuable insights into patient experiences and treatment satisfaction, although the reliability and accuracy of self-reported data needs to be carefully considered.
Data Cleaning and Preprocessing Steps
Data cleaning is the crucial first step, addressing issues like missing values, outliers, and inconsistencies. Missing values can be handled through imputation techniques, such as mean/median imputation or more sophisticated methods like k-nearest neighbors imputation. Outliers, which can significantly skew results, require careful consideration; they may represent genuine extreme cases or data entry errors. Inconsistencies in data formats and terminology need to be standardized for consistency. For instance, different clinics might use different codes for the same acupuncture point. A standardized coding system needs to be implemented to ensure uniformity.
Data Transformation and Feature Scaling
After cleaning, data transformation is necessary to prepare the data for machine learning algorithms. This may involve converting categorical variables (e.g., acupuncture point location) into numerical representations using techniques like one-hot encoding. Continuous variables may require transformations to achieve normality or to address skewness, using techniques like logarithmic or Box-Cox transformations. Feature scaling ensures that features with different scales do not disproportionately influence the machine learning model. Common scaling techniques include standardization (z-score normalization) and min-max scaling. For example, standardizing the duration of acupuncture treatment ensures that this variable is weighted equally alongside other variables, such as patient age, which might have a vastly different scale.
Data Privacy and Security
Protecting patient privacy and data security is paramount in acupuncture research involving machine learning. This requires adherence to relevant regulations, such as HIPAA in the United States and GDPR in Europe. Data anonymization and de-identification techniques should be employed to remove or mask any personally identifiable information. Secure data storage and access control mechanisms are essential to prevent unauthorized access and data breaches. Data encryption both in transit and at rest is critical for protecting sensitive patient information. Furthermore, informed consent from participants is crucial, clearly outlining how their data will be used and protected. For instance, all identifying information such as names and addresses can be replaced with unique identifiers. The use of differential privacy techniques can further enhance data privacy by adding carefully calibrated noise to the data, while still allowing for meaningful analysis.
Visualizing Acupuncture Treatment Effects with Machine Learning
Unlocking the secrets of acupuncture’s effectiveness often involves navigating a complex interplay of factors. Visualizing this data, however, can transform raw numbers into insightful narratives, revealing patterns and trends that might otherwise remain hidden. Machine learning empowers us to create sophisticated visualizations that not only present this data clearly but also uncover previously unseen relationships between acupuncture point stimulation, patient characteristics, and treatment outcomes.
Visualizing the effects of acupuncture treatment using machine learning allows for a deeper understanding of its mechanisms and efficacy. By leveraging the power of data visualization, we can transform complex datasets into easily interpretable insights, fostering better communication and collaboration among researchers, clinicians, and patients. This enhanced understanding can ultimately lead to more personalized and effective acupuncture treatments.
Data Visualization Techniques for Acupuncture Research
Several visualization methods effectively represent the relationships between acupuncture point stimulation, patient characteristics, and treatment outcomes. These techniques allow researchers to explore the data, identify patterns, and communicate findings in a clear and compelling manner.
- Scatter Plots: Scatter plots can illustrate the correlation between two continuous variables, such as the intensity of acupuncture stimulation (measured, for example, by the depth of needle insertion or electrical stimulation parameters) and the reduction in pain scores. For instance, a positive correlation would show that higher stimulation intensity is associated with greater pain relief. Each point on the scatter plot represents a single patient, allowing for visual identification of outliers or subgroups responding differently to treatment.
- Heatmaps: Heatmaps are ideal for visualizing the relationship between multiple variables. Imagine a heatmap where rows represent different acupuncture points, columns represent patient characteristics (e.g., age, gender, disease severity), and the color intensity reflects the treatment outcome (e.g., pain reduction, improvement in range of motion). A darker shade might indicate a stronger positive effect of a specific acupuncture point on a particular patient group.
- Network Graphs: These can visually represent the interconnectedness of acupuncture points within meridians and their influence on treatment outcomes. Nodes could represent acupuncture points, and the edges could represent the strength of their interaction, based on the observed treatment effects. The thickness of the edges could reflect the magnitude of the effect. This approach can help identify key acupuncture points within a meridian or network that significantly contribute to the overall treatment effect.
Interactive Visualization of Meridian Effects
Creating an interactive visualization to explore the effects of acupuncture on different meridians offers a dynamic way to understand complex relationships. This type of visualization would allow users to select specific meridians, explore the associated acupuncture points, and observe the treatment outcomes for various patient subgroups. For example, an interactive dashboard could display heatmaps for each meridian, showing the effectiveness of points along that meridian for different conditions. Users could then filter the data based on patient characteristics (age, gender, etc.) and observe how the treatment effects vary across different groups. This interactive approach enhances data exploration and promotes a deeper understanding of the meridian system’s role in acupuncture therapy.
Identifying Patterns and Trends in Large Acupuncture Datasets
Machine learning algorithms are powerful tools for analyzing large acupuncture datasets and identifying patterns and trends that may not be apparent through traditional statistical methods. For example, clustering algorithms can group patients with similar responses to acupuncture treatment, revealing subgroups that might benefit from specific acupuncture protocols. Classification algorithms can predict treatment outcomes based on patient characteristics and acupuncture point selection, potentially personalizing treatment strategies. Regression models can quantify the relationship between acupuncture stimulation parameters and treatment outcomes, providing valuable insights into the mechanisms of acupuncture therapy. By applying these techniques, researchers can gain a more comprehensive understanding of acupuncture’s effectiveness and optimize treatment strategies for different patient populations. For instance, a study might use a random forest model to predict the likelihood of successful pain management based on factors such as patient age, gender, disease severity, and the specific acupuncture points used.
Study Design Considerations for Machine Learning in Acupuncture Research
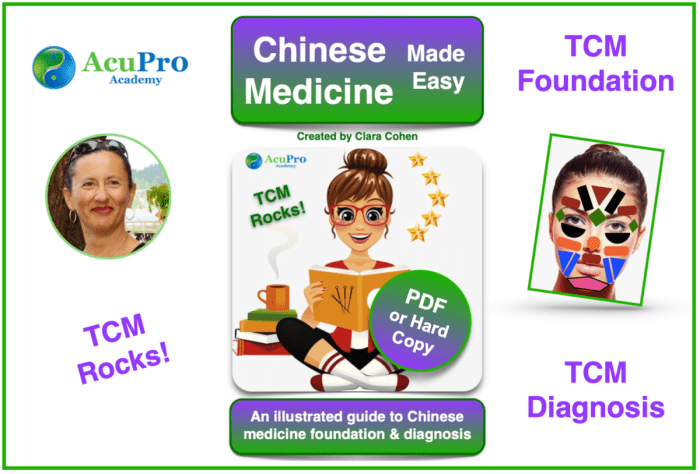
Source: acuproacademy.com
Designing a clinical trial that effectively leverages machine learning to investigate acupuncture’s efficacy requires meticulous planning. This involves not only establishing a robust study design but also addressing potential confounding factors and navigating the ethical and regulatory landscape of AI-driven healthcare research. This section details a sample study design and highlights key considerations.
A Clinical Trial Design: Patient Stratification with Machine Learning
This study will investigate the effectiveness of acupuncture for chronic low back pain (CLBP), a prevalent condition amenable to acupuncture treatment. The study will employ machine learning to stratify patients into subgroups predicted to respond differently to acupuncture, optimizing treatment allocation and enhancing outcome prediction.
The study will employ a randomized controlled trial (RCT) design. Participants with CLBP, meeting predefined inclusion criteria (e.g., pain duration, intensity, and diagnostic imaging results), will be recruited. Pre-treatment data, including demographics, medical history, pain scores (e.g., Visual Analog Scale – VAS), and relevant biomarkers (e.g., inflammatory markers), will be collected. This data will be used to train a machine learning model (e.g., a support vector machine or random forest) to predict treatment response. This model will stratify patients into high-response and low-response groups.
Patients will then be randomly assigned to either acupuncture treatment or a sham acupuncture control group, stratified by their predicted response. The primary outcome measure will be pain reduction at a specified follow-up period (e.g., 8 weeks), measured using VAS. Secondary outcome measures may include functional improvement (e.g., Oswestry Disability Index), quality of life assessments, and changes in biomarkers. The effectiveness of the machine learning-based stratification will be evaluated by comparing treatment outcomes between the stratified groups. Statistical analyses will determine if the model accurately predicted treatment response and whether the stratification strategy improved treatment efficacy.
Potential Confounding Factors, Machine learning chinese medicine acupuncture study design
Several factors could confound the results of this study. These include patient characteristics (age, gender, comorbidities, prior treatment history), practitioner experience, acupuncture point selection, and variations in treatment protocols. To mitigate these, rigorous standardization of treatment procedures, careful patient selection based on strict inclusion/exclusion criteria, and statistical adjustment for confounding variables during data analysis are crucial. For example, the experience of the acupuncturist could be considered as a covariate in the analysis, allowing for a more accurate assessment of the treatment effect. Furthermore, detailed documentation of all acupuncture points used and the treatment parameters (needle depth, retention time, stimulation technique) will minimize variability.
Ethical Considerations and Regulatory Requirements
Ethical considerations are paramount in this type of research. Informed consent is essential, ensuring participants understand the study’s purpose, procedures, risks, and benefits. Data privacy and security must be ensured through anonymization and secure data storage, complying with relevant data protection regulations (e.g., GDPR, HIPAA). The study protocol will need to be reviewed and approved by an Institutional Review Board (IRB) or Ethics Committee before commencement. Regulatory requirements for conducting clinical trials, including those involving AI algorithms, must be strictly adhered to. Transparency regarding the machine learning model, including its training data, algorithms, and performance metrics, is crucial for reproducibility and validation. The potential for bias in the algorithm should be addressed through rigorous testing and validation procedures.
Outcome Summary: Machine Learning Chinese Medicine Acupuncture Study Design
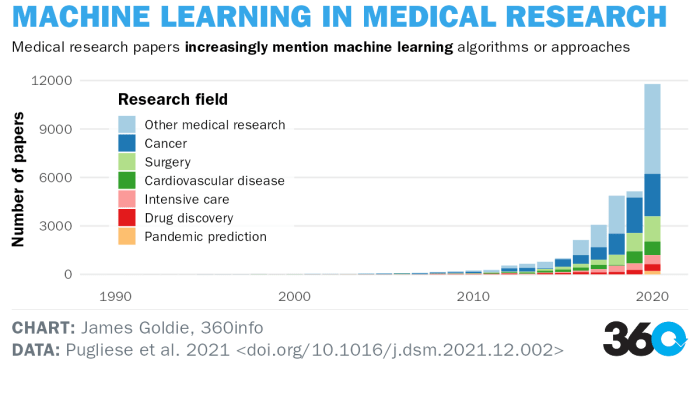
Source: amazonaws.com
The integration of machine learning into Chinese medicine acupuncture research holds immense potential. By leveraging the power of data analysis and sophisticated algorithms, we can move beyond traditional approaches and unlock a new era of personalized and evidence-based acupuncture. While challenges remain in data acquisition, ethical considerations, and algorithm development, the possibilities are truly exciting. This study design framework offers a roadmap for future research, paving the way for a more precise, effective, and scientifically validated approach to acupuncture treatment. The future of acupuncture is data-driven, and it’s only just beginning.