The Boring Future of Generative AI: We’ve all been promised a world transformed by AI – self-driving cars, personalized medicine, robots doing our chores. But what if the reality is… less exciting? What if the future of generative AI is, well, kinda boring? This isn’t about killer robots or sentient machines; it’s about the subtle, often overlooked, ways AI might subtly reshape our lives, not with a bang, but a whimper.
This piece dives into the chasm between the hyped-up promises of generative AI and its current, often underwhelming, reality. We’ll explore the technological hurdles, ethical dilemmas, and economic impacts, painting a picture of a future where AI is more of a helpful assistant than a revolutionary force. Prepare for a dose of realistic, perhaps even disappointing, truth about the future of AI.
The Current State of Generative AI
Generative AI, the exciting field of artificial intelligence focused on creating new content, is currently experiencing a period of rapid advancement and equally rapid disillusionment. While the technology shows incredible promise, its limitations are equally striking, leading to both spectacular successes and spectacular failures. Understanding the current state requires a balanced look at its capabilities and shortcomings.
Generative AI excels at tasks involving pattern recognition and synthesis. It can generate realistic images, write coherent text, compose music, and even create functional code. However, these capabilities are often constrained by the data it’s trained on and the inherent limitations of the underlying algorithms. The output, while sometimes impressive, can also be nonsensical, biased, or factually inaccurate.
Capabilities and Limitations of Generative AI Technologies
Generative AI models, primarily large language models (LLMs) and diffusion models, demonstrate impressive abilities in content creation across various modalities. LLMs can generate human-quality text for tasks like writing summaries, translating languages, and answering questions. Diffusion models excel at generating high-resolution images from text prompts. However, these models often struggle with nuanced understanding, logical consistency, and factual accuracy. Their output can be highly sensitive to subtle changes in input, and they may exhibit biases reflected in the training data. Furthermore, generating truly novel and creative content, rather than simply recombining existing patterns, remains a significant challenge. The “creative spark” is still largely absent.
Successful and Unsuccessful Applications of Generative AI
Successful applications include the use of generative AI in marketing for creating engaging ad copy and visuals, its use in software development for assisting with code generation, and its use in the entertainment industry for generating unique game assets and storylines. Unsuccessful applications are often found where nuanced understanding or factual accuracy is crucial. For example, relying solely on generative AI for medical diagnosis or legal advice would be incredibly risky due to its potential for errors and hallucinations. Similarly, using it to generate news articles without thorough fact-checking can lead to the spread of misinformation.
Instances Where Generative AI Has Fallen Short of Expectations
One notable instance is the tendency of generative AI models to “hallucinate” – fabricating information that sounds plausible but is entirely false. This has been observed in various applications, from generating fictional news articles to creating historical summaries. Another area where expectations have not been met is the difficulty in controlling the style and tone of the generated content. While users can provide prompts, achieving consistent and predictable results remains a significant challenge. Finally, the ethical concerns surrounding bias and potential misuse of the technology continue to hinder its widespread adoption.
Technological Hurdles Preventing Widespread Generative AI Adoption
Several key technological hurdles remain. The computational resources required to train and run these models are substantial, limiting access for many researchers and organizations. Furthermore, ensuring the ethical use of generative AI, addressing biases in training data, and mitigating the risk of misuse require ongoing research and development. The lack of standardized evaluation metrics and the difficulty in assessing the reliability and trustworthiness of generated content also pose significant challenges to widespread adoption. The energy consumption associated with training these large models is also a significant concern.
Over-Hype and Under-Delivery
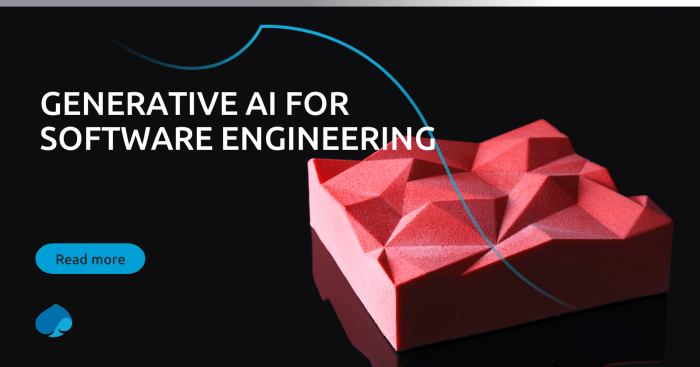
Source: capgemini.com
Generative AI has exploded onto the scene, promising a revolution in everything from art and writing to scientific research and business processes. But the reality, as always with emerging technologies, is a bit more nuanced. The chasm between the breathless hype surrounding generative AI and its actual, tangible impact is widening, leaving many feeling a little…underwhelmed. Let’s dive into why the promise hasn’t quite met the performance.
The media, naturally, loves a good story. And the narrative of AI creating masterpieces overnight, replacing human workers en masse, and solving all our problems is undeniably compelling. However, this portrayal often ignores the limitations and complexities inherent in the technology. The result? Unrealistic expectations, leading to disappointment when the reality falls short. This isn’t to say generative AI is worthless; it’s simply not the magic bullet many believed it to be.
Unfulfilled Promises in Specific Areas
Many predicted that generative AI would rapidly automate entire industries. While it’s improved efficiency in some areas, the complete automation of complex tasks like legal document review or medical diagnosis remains elusive. The technology excels at specific, well-defined tasks, but struggles with the nuance, ambiguity, and contextual understanding required for broader applications. For example, while AI can generate impressive-looking marketing copy, it often lacks the creative spark and strategic insight of a human copywriter. Similarly, AI-generated artwork, while visually stunning at times, often lacks the emotional depth and conceptual originality of human artists. The expectation of fully autonomous systems capable of handling multifaceted real-world scenarios has not been met, at least not yet.
The Reality Check: Progress vs. Predictions
Early predictions painted a picture of fully autonomous, general-purpose AI within a few years. Think self-driving cars navigating complex urban environments flawlessly, or AI doctors diagnosing illnesses with perfect accuracy. The reality is far more incremental. Self-driving technology, for instance, is still under heavy development, facing numerous challenges in handling unpredictable situations. While AI has made impressive strides in medical image analysis, it’s still primarily used as a supportive tool for human doctors, not a replacement. The timeline for achieving truly general-purpose AI remains uncertain, with experts offering widely varying estimates. The hype cycle, fueled by sensationalized media coverage, created a distorted sense of the technology’s maturity and capabilities, leading to a significant gap between expectation and reality.
Ethical Concerns and Societal Impact
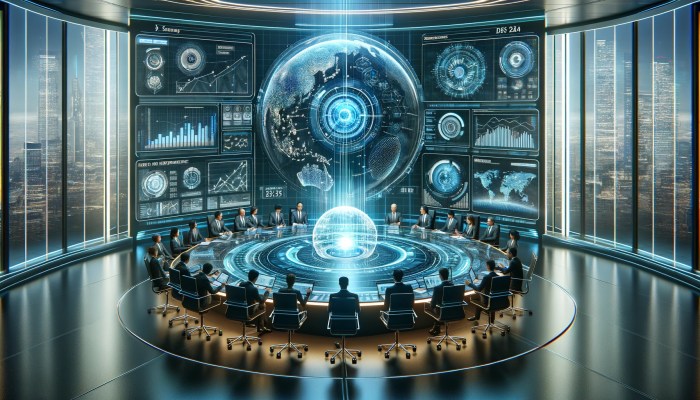
Source: unite.ai
Yeah, so generative AI? Kinda getting stale, right? All the hype, but where’s the real innovation? Ironically, a glimmer of exciting potential lies in unexpected places, like advancements in future video games visual accessibility , which could actually breathe some life into the whole thing. But let’s be real, even that might eventually succumb to the same predictable patterns.
The boring future of generative AI looms large.
Generative AI, while promising incredible advancements, presents a Pandora’s Box of ethical dilemmas and potential societal disruptions. Its power to create realistic content – from text and images to audio and video – raises serious questions about authenticity, accountability, and the very fabric of our information ecosystem. Ignoring these concerns risks a future where trust erodes, misinformation proliferates, and the line between reality and fabrication blurs beyond recognition.
A Scenario of Malicious Use
Imagine a sophisticated deepfake video, indistinguishable from reality, depicting a prominent political figure admitting to a serious crime. This video, generated using advanced generative AI, could be released strategically just before a crucial election, causing widespread chaos and potentially influencing the outcome. The perpetrator remains anonymous, leaving no clear trail of accountability, and the damage to public trust is irreparable. This scenario highlights the potential for generative AI to be weaponized for political manipulation, social unrest, or even financial fraud, creating a world where truth becomes a subjective and easily manipulated commodity.
Potential Negative Societal Impacts
The widespread adoption of generative AI carries several risks to society. These impacts aren’t simply theoretical; we’re already seeing early signs in the current media landscape.
- Increased Misinformation and Disinformation: The ease with which generative AI can create convincing fake news articles, images, and videos makes it a potent tool for spreading misinformation, eroding public trust in legitimate sources of information.
- Job Displacement: As generative AI becomes more capable, it threatens to automate tasks currently performed by human workers in various creative and writing-related professions, leading to job losses and economic disruption.
- Bias Amplification: Generative AI models are trained on vast datasets that may reflect existing societal biases. This can lead to AI systems perpetuating and even amplifying harmful stereotypes and prejudices in their outputs.
- Privacy Violations: The use of personal data to train generative AI models raises concerns about privacy violations and the potential for misuse of sensitive information.
- Copyright Infringement: The ability of generative AI to create content that mimics existing works raises complex legal and ethical questions regarding copyright ownership and intellectual property rights.
Benefits and Drawbacks of Generative AI Development
The development of generative AI presents a complex trade-off between potential benefits and significant drawbacks. Weighing these carefully is crucial for responsible innovation.
Benefits | Drawbacks |
---|---|
Increased efficiency and productivity in various industries | Potential for widespread job displacement and economic inequality |
Creation of novel and innovative content in art, design, and entertainment | Risk of increased misinformation and manipulation |
Advancements in scientific research and technological development | Ethical concerns regarding bias, privacy, and accountability |
Personalized learning experiences and accessibility improvements | Potential for misuse in malicious activities like deepfakes |
Ethical Dilemmas in Creative Fields
The application of generative AI in creative fields presents a unique set of ethical challenges. Consider the question of authorship: if an AI generates a piece of art or music, who owns the copyright? Is it the programmer who created the AI, the user who prompted the AI, or the AI itself? Furthermore, the potential for AI to mimic the style of existing artists raises concerns about originality and plagiarism. The very definition of artistic creation is being challenged, leading to debates about authenticity, originality, and the role of human creativity in a world increasingly shaped by artificial intelligence. These questions require careful consideration and a nuanced approach to ensure the ethical and responsible use of generative AI in creative endeavors.
Economic and Job Market Implications

Source: slidegeeks.com
Generative AI is poised to reshape the economic landscape, impacting various industries and the job market in profound ways. While offering potential for increased efficiency and productivity, it also presents significant challenges related to job displacement and the need for workforce adaptation. Understanding these implications is crucial for navigating the transition to a future shaped by this powerful technology.
The integration of generative AI will vary significantly across industries. Some sectors will experience more dramatic transformations than others, leading to a complex and uneven distribution of both opportunities and challenges. The speed of adoption will also play a crucial role, with early adopters potentially gaining a competitive edge while others struggle to keep pace.
Impact on Various Industries and Job Sectors
Generative AI’s impact will be felt across numerous sectors. In customer service, AI-powered chatbots are already replacing human agents for routine inquiries. Marketing and advertising will see increased automation of content creation, potentially reducing the need for human copywriters and designers. Manufacturing could see increased automation of design and production processes, impacting roles in engineering and assembly. The legal profession might see AI assisting with document review and contract analysis, potentially altering the roles of paralegals and junior lawyers. The healthcare industry could see AI used for drug discovery and personalized medicine, changing the roles of researchers and medical professionals. These are just a few examples of how generative AI is likely to impact various industries and the jobs within them. The extent of this impact will depend on factors such as the rate of technological advancement and the willingness of businesses to adopt these technologies.
Examples of Jobs Affected by Generative AI, The boring future of generative ai
Several job roles are particularly vulnerable to automation by generative AI. Data entry clerks, whose work involves repetitive tasks easily handled by AI, are a prime example. Similarly, telemarketers and other customer service representatives handling routine inquiries are at risk. In creative industries, roles like graphic designers and writers could see significant changes as AI tools become more sophisticated. However, it’s important to note that many jobs will not be entirely replaced, but rather augmented by AI, leading to changes in job responsibilities and required skills. For example, while AI can generate basic marketing copy, human marketers will still be needed for strategic planning and creative direction.
Strategies for Workforce Adaptation
Adapting to the changing landscape of generative AI requires a multifaceted approach. Investing in education and training programs that focus on skills complementary to AI is crucial. This includes upskilling and reskilling initiatives that equip workers with the technical and analytical abilities needed to work alongside AI systems. Furthermore, fostering a culture of lifelong learning is essential, enabling workers to adapt to evolving job requirements. Government policies also play a vital role, supporting retraining programs and providing social safety nets for those displaced by automation. Businesses should also actively participate by investing in employee training and creating opportunities for workers to develop new skills.
Economic Benefits and Drawbacks of Generative AI Adoption
Widespread adoption of generative AI holds the potential for significant economic benefits, including increased productivity, reduced costs, and the creation of new industries and jobs. However, it also presents potential drawbacks. Job displacement due to automation could lead to increased unemployment and income inequality if not managed effectively. The concentration of power in the hands of a few companies controlling advanced AI technologies is another potential concern. Moreover, ethical considerations, such as bias in AI algorithms and the potential for misuse, need to be carefully addressed to ensure responsible and equitable deployment of this technology. The overall economic impact will depend on how effectively societies and economies adapt to the changes brought about by generative AI.
Technological Limitations and Future Directions: The Boring Future Of Generative Ai
Generative AI, despite its impressive feats, remains hobbled by several key limitations. While it can produce remarkably human-like text, images, and even code, its capabilities are far from boundless. Understanding these limitations is crucial for shaping realistic expectations and guiding future research. The path towards truly intelligent and creative AI systems is paved with significant challenges, but also exciting possibilities.
The current generation of generative AI models relies heavily on massive datasets for training. This dependence creates several bottlenecks. First, the sheer volume of data required is computationally expensive and environmentally unsustainable. Second, biases present in the training data inevitably propagate into the generated outputs, leading to ethical concerns and potentially harmful societal impacts. Third, these models often struggle with tasks requiring genuine understanding and reasoning beyond pattern recognition. They excel at mimicking patterns but often lack the underlying comprehension.
Data Dependency and Bias Mitigation
Over-reliance on massive datasets introduces inherent biases. For example, a model trained on a dataset predominantly featuring images of a certain ethnicity might generate outputs that consistently reflect that bias. Mitigating this requires careful curation of training data, employing techniques like data augmentation and adversarial training to reduce biases, and developing methods for detecting and correcting biases in the generated outputs. Imagine a facial recognition system trained on predominantly light-skinned faces; its performance on darker skin tones would likely be significantly degraded, highlighting the importance of diverse and representative datasets. Future advancements might involve developing models that can learn effectively from smaller, more curated datasets, reducing the environmental and ethical concerns associated with massive data consumption.
Creativity and Unpredictability
Creating truly creative and unpredictable AI systems is a significant challenge. Current generative models primarily operate by statistically predicting the most probable next element in a sequence. While this can produce impressive results, it lacks the element of genuine surprise or originality that characterizes human creativity. The inherent randomness of human thought processes, driven by factors beyond simple statistical probabilities, remains a significant hurdle for AI. Consider the difference between a computer-generated poem that adheres to established poetic structures and a poem that breaks those structures in a surprising and meaningful way – that’s the gap generative AI currently struggles to bridge. Future research might explore incorporating elements of randomness and exploration into model architectures, potentially drawing inspiration from biological systems like the human brain.
Improved Reasoning and Contextual Understanding
Current generative AI models often struggle with tasks requiring genuine reasoning and contextual understanding. They can process information, but their ability to integrate information across different contexts or reason logically about complex situations remains limited. For instance, a model might generate grammatically correct text that is factually inaccurate or nonsensical because it fails to grasp the underlying context. Advancements in areas like knowledge representation, reasoning, and common sense reasoning are critical to overcome this limitation. This could involve integrating symbolic reasoning techniques with deep learning models or developing models that can explicitly represent and reason with knowledge graphs. This would enable AI systems to understand the relationships between different pieces of information and use that understanding to generate more accurate and insightful outputs. For example, a model that understands the relationship between “rain” and “wet pavement” would be less likely to generate a scenario where it’s raining but the pavement is dry.
Enhanced Explainability and Control
The “black box” nature of many generative AI models presents another significant challenge. Understanding how these models arrive at their outputs is crucial for ensuring reliability, trustworthiness, and accountability. The lack of explainability makes it difficult to identify and correct errors or biases. Research into developing more explainable AI models is vital. This could involve techniques like attention mechanisms that highlight the parts of the input that most influenced the output, or the development of models with simpler architectures that are easier to interpret. Improved control over the generative process is also important, allowing users to guide the model’s output more effectively and ensure it aligns with their specific needs and intentions. Imagine being able to specify constraints or preferences that guide the model’s creativity without entirely dictating the outcome.
The Role of Data and Bias
Generative AI models, despite their impressive capabilities, are fundamentally shaped by the data they are trained on. This data, often vast and complex, acts as the blueprint for the AI’s behavior and output. Consequently, biases present in this training data inevitably seep into the AI’s responses, leading to potentially harmful and discriminatory outcomes. Understanding this relationship between data and bias is crucial for developing responsible and equitable AI systems.
The problem stems from the fact that training datasets rarely reflect the true diversity and complexity of the real world. They may overrepresent certain demographics or viewpoints while underrepresenting others, creating a skewed perspective that the AI system internalizes. This skewed perspective can manifest in various ways, from subtle biases in language generation to outright discriminatory actions in decision-making systems. For instance, an AI trained on a dataset predominantly featuring male faces might struggle to accurately identify female faces, highlighting the critical need for diverse and representative datasets.
Biased Datasets and Harmful Stereotypes
Biased datasets frequently perpetuate harmful stereotypes and misinformation. If a generative AI model is trained on text data containing racist or sexist language, it is likely to reproduce similar biases in its own output. This can lead to the reinforcement of harmful stereotypes and the spread of misinformation, impacting vulnerable groups and undermining social equity. For example, an AI trained on news articles that consistently portray a certain ethnic group in a negative light might generate text that perpetuates these negative stereotypes, thereby contributing to real-world discrimination. Similarly, a model trained on biased medical data might misdiagnose patients from underrepresented groups, leading to potentially harmful consequences.
Strategies for Mitigating Bias in Generative AI
Addressing bias in generative AI requires a multi-faceted approach. It’s not simply a matter of “fixing” the data, but rather a continuous process of careful consideration and refinement throughout the entire AI lifecycle. Effective strategies are needed to ensure fairness and mitigate the potential for harm.
- Data Augmentation and Balancing: Actively seeking out and incorporating underrepresented data points to create a more balanced training dataset. This involves strategies like oversampling minority groups or synthetic data generation techniques to balance the representation of different demographics.
- Bias Detection and Mitigation Tools: Utilizing algorithms and tools designed to identify and quantify biases present in datasets. These tools can help pinpoint areas where the data is skewed and inform strategies for mitigation.
- Algorithmic Fairness Techniques: Implementing algorithmic techniques that actively mitigate bias during the model training process. This could involve using fairness-aware loss functions or post-processing techniques to adjust model outputs.
- Human-in-the-Loop Systems: Integrating human oversight and feedback into the development and deployment of generative AI systems. Human review can help identify and correct biases that may be missed by automated methods.
- Diverse and Inclusive Development Teams: Ensuring that the teams developing and deploying generative AI systems are diverse and inclusive. Diverse perspectives are crucial for identifying and addressing potential biases throughout the development process.
Examples of Unfair or Discriminatory Outcomes
The consequences of bias in generative AI can be far-reaching and impactful. Consider the following examples:
* Recruitment AI: An AI system trained on biased data from past hiring practices might inadvertently discriminate against certain demographic groups, perpetuating existing inequalities in the workplace.
* Loan Applications: A biased AI used to assess loan applications could unfairly deny loans to individuals from underrepresented communities based on biased historical data.
* Facial Recognition Technology: Facial recognition systems trained on datasets lacking diversity have demonstrated significantly lower accuracy rates for individuals with darker skin tones, leading to misidentification and potential miscarriages of justice.
* Healthcare Diagnosis: As previously mentioned, biased medical data can lead to inaccurate diagnoses and unequal access to quality healthcare for certain groups.
Illustrative Examples
So, the hype train has slowed, the singularity hasn’t happened, and generative AI is… well, kind of boring. Let’s paint a picture of a future where these tools are ubiquitous but unremarkable, a future where the revolutionary has become the routine.
The sheer pervasiveness of these tools is precisely what makes this future so mundane. Instead of groundbreaking advancements, we see incremental improvements, a slow creep of automation that subtly alters, but doesn’t dramatically reshape, our lives.
A Typical Workday in the Age of Uninspired AI
Imagine waking up to a smart home system, powered by a generative AI that optimizes your morning routine based on your past habits. Not a personalized, insightful optimization, mind you, but a slightly faster, slightly more efficient version of what you already do. Your AI-generated breakfast suggestion? The same oatmeal you’ve had for the past three months, but with a slightly different recipe generated from a database of bland breakfast options. Your commute is “optimized” by an AI that chooses the slightly less congested route, saving you maybe two minutes. At work, your AI assistant generates reports that are technically correct but utterly devoid of insightful analysis, merely regurgitating data in a slightly different format. Lunch is a predictably bland AI-generated meal suggestion from the same three restaurants you always order from. The workday ends with an AI-generated summary of your accomplishments that feels utterly hollow, failing to capture the nuances and complexities of your actual contributions. The evening follows the same predictable pattern: AI-curated entertainment, AI-optimized sleep schedule, and the quiet hum of technology that never truly surprises or excites. The overall feeling is one of efficient, predictable, and ultimately, unfulfilling sameness.
Homogenization of Creative Outputs
The ease of access to generative AI tools poses a significant threat to originality and diversity in creative fields. The algorithm’s reliance on existing data leads to a predictable outcome: the replication and recombination of familiar styles and ideas.
The ease with which anyone can generate passable content, without the need for specialized skills or extensive training, contributes to an overwhelming flood of similar products. This makes it difficult for truly original work to stand out and be noticed.
- Music: A landscape dominated by AI-generated tracks that sound remarkably similar, lacking the unique nuances of human expression and emotional depth.
- Visual Arts: An overabundance of AI-generated images that mimic existing styles and trends, lacking originality and emotional impact.
- Literature: A sea of AI-generated novels and short stories that adhere to predictable plot structures and character archetypes, lacking the depth and complexity of human storytelling.
- Film: AI-generated scripts and storyboards that lead to a predictable and uninspired cinematic landscape.
Limited vs. Significant Generative AI Progress
A future with limited generative AI progress would see these tools integrated into everyday life in a subtle, almost imperceptible way. Think of it as the slow, steady evolution of existing technologies, like the gradual improvement of word processors or search engines. It would lead to increased efficiency in certain tasks, but not a fundamental shift in how we live or work. In contrast, a future with significant advancements might lead to the automation of complex jobs, creative breakthroughs that redefine industries, and potentially even new forms of human-computer interaction. However, this future also carries significant risks, including widespread job displacement and the potential for misuse of powerful AI systems. The key difference lies in the transformative potential: limited progress leads to incremental improvements, while significant progress might lead to revolutionary change—with all the accompanying uncertainties.
End of Discussion
So, is the future of generative AI truly boring? Maybe. But “boring” doesn’t necessarily mean “bad.” It means we need to temper expectations, focus on responsible development, and understand the limitations of the technology. Instead of fantasizing about sentient robots, let’s focus on using AI to solve real-world problems, improve efficiency, and enhance – not replace – human creativity. The future might not be filled with dramatic breakthroughs, but it could be a future where AI quietly, and perhaps a little boringly, makes our lives a little better.