Spotify AI music robot listeners: Ever wondered how Spotify magically knows what song you’ll crave next? It’s not magic, it’s a complex AI system fueled by your listening habits and, surprisingly, legions of digital “robot listeners.” These aren’t your average bots; they’re sophisticated algorithms designed to refine Spotify’s recommendation engine, shaping the music landscape and influencing what millions hear daily. This deep dive explores the fascinating world of Spotify’s AI, its robot listeners, and the ethical implications of algorithmic music curation.
We’ll dissect Spotify’s AI architecture, examining the data points it uses – from your genre preferences to your listening time of day – to personalize your experience. We’ll also investigate the role of these “robot listeners” in improving the algorithm’s accuracy and uncover the potential biases and limitations of AI-driven music discovery. Get ready to uncover the secrets behind your personalized Spotify experience.
Spotify’s AI Music Recommendation System
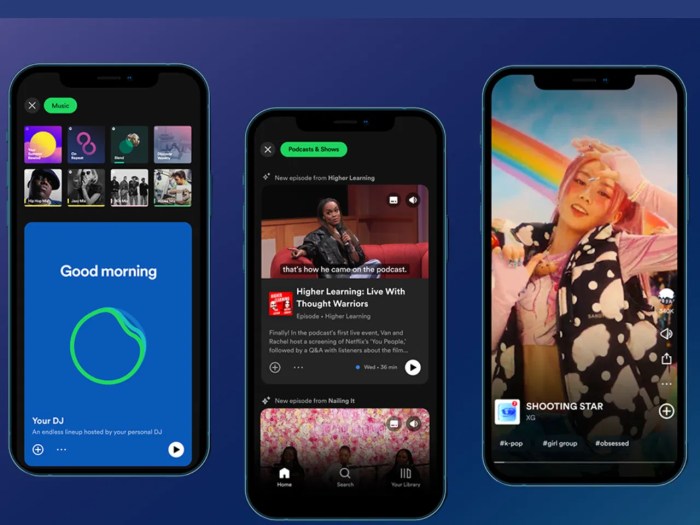
Source: news9live.com
Spotify’s AI-powered music recommendation engine is a complex system that leverages vast amounts of data to personalize the listening experience for millions of users. It’s not just about playing songs you’ve liked before; it’s about predicting what you might like next, based on a sophisticated understanding of your musical tastes and listening habits. This system is constantly evolving, learning, and refining its predictions to ensure a consistently engaging user experience.
System Architecture
Spotify’s recommendation system isn’t a single algorithm but rather a combination of various machine learning models working in concert. These models analyze diverse data points to generate personalized recommendations. A key component is collaborative filtering, which identifies users with similar listening habits and recommends music that those similar users enjoy. Content-based filtering also plays a crucial role, recommending songs similar to those a user has already listened to or liked. Beyond these core methods, Spotify employs more advanced techniques like deep learning models to capture nuanced patterns in user behavior and musical characteristics. The system is continuously updated and improved through A/B testing and real-time feedback.
Data Points Used for Personalization
The system’s effectiveness stems from its ability to gather and analyze a wide array of data. This includes explicit user data, such as songs liked, playlists created, and artists followed. Implicit data, which is gathered passively, is equally crucial. This includes listening history (songs played, skipped, and the order in which they were played), duration of listening sessions, time of day of listening, and even device used. Beyond individual user data, the system also leverages data about the songs themselves – genre, tempo, instrumentation, lyrical content, and even the acoustic characteristics of the recording. This holistic approach allows for a deeply personalized experience.
Influence of User Listening Habits
User listening habits are the lifeblood of Spotify’s recommendation system. The algorithm doesn’t just track what you listen to; it analyzes *how* you listen. Repeated plays of a particular song indicate strong preference. Skipping a song early suggests a lack of interest. The order in which you listen to songs reveals potential patterns and transitions in your musical tastes. For instance, if a user consistently listens to a certain artist’s albums in order, the system might prioritize recommending the next album in the sequence. The system is constantly learning from these subtle cues to refine its understanding of individual preferences.
Comparison with Other Music Streaming Services
While other music streaming services like Apple Music, YouTube Music, and Amazon Music also employ AI-driven recommendation systems, Spotify’s is generally considered one of the most sophisticated and effective. The key differentiators often lie in the scale of data Spotify possesses, the complexity of its algorithms, and the level of personalization it offers. While direct comparisons are difficult due to the proprietary nature of these systems, Spotify’s reputation for strong recommendations is widely recognized within the industry.
Hypothetical User Profile and Tailored Recommendations
Let’s consider a hypothetical user, “Sarah,” a 28-year-old who enjoys indie pop, alternative rock, and occasionally listens to classic rock. Her listening history shows frequent plays of artists like The 1975, Lorde, and Arctic Monkeys, with occasional forays into Fleetwood Mac and The Rolling Stones. Based on this profile, Spotify’s algorithm would likely recommend new releases from similar indie pop and alternative rock artists, perhaps suggesting artists with similar musical styles or even playlists curated around these genres. It might also introduce her to lesser-known artists within these genres, based on collaborative filtering and content-based recommendations. The algorithm could also identify the occasional classic rock listening and subtly incorporate similar artists into its recommendations, showcasing the system’s ability to navigate a user’s diverse tastes. The recommendations would be dynamic, adapting and evolving as Sarah’s listening habits change over time.
The Role of “Robot Listeners” in Spotify’s AI
Spotify’s AI-powered music recommendation system isn’t just fueled by your listening habits; it also relies heavily on a network of “robot listeners”—sophisticated algorithms designed to mimic human behavior and preferences. These digital entities play a crucial, often unseen, role in refining the system’s accuracy and expanding its understanding of musical tastes.
The purpose of these simulated listeners is multifaceted. They act as a crucial testing ground for new algorithms and features, allowing developers to evaluate their impact without needing millions of real users to participate in extensive A/B testing. They can listen to and analyze vast amounts of music, far exceeding the capacity of any human, providing a richer dataset for the AI to learn from. By exposing the system to a diverse range of musical styles and genres, these “robot listeners” help prevent biases that might emerge from relying solely on real-world user data.
Simulated Listener Functionality in Refining Recommendations
Robot listeners are programmed with diverse musical profiles, simulating various demographics, listening habits, and preferences. This allows Spotify to test how different algorithms perform across a wide spectrum of potential users. For example, one simulated listener might mimic a hardcore metal fan, while another might represent a casual listener who enjoys a wide variety of genres. By analyzing how the algorithm responds to each simulated listener, developers can identify and address weaknesses in the system’s ability to provide personalized recommendations. The data generated by these simulated listeners provides a controlled environment for testing and iterative improvement of the recommendation engine.
Ethical Considerations of Simulated Listeners
The use of simulated listeners raises some ethical questions. While they don’t have feelings or personal preferences in the same way humans do, their role in shaping musical recommendations indirectly influences what music is promoted and discovered. There’s a potential for algorithmic bias if the simulated listeners aren’t carefully designed to represent a truly diverse range of tastes and preferences. This could lead to certain genres or artists being unfairly favored or marginalized, ultimately impacting the cultural landscape of music discovery. Transparency regarding the use of these simulated listeners and the measures taken to mitigate bias is therefore crucial.
Real User Data vs. Simulated Listener Data
While real user data is invaluable for understanding actual listening habits, it has limitations. Real user data is often sparse, noisy, and prone to biases based on existing popularity. Simulated listener data, on the other hand, provides a clean, controlled dataset that can be used to test the algorithm’s robustness and identify areas for improvement. The ideal approach is a hybrid model that combines both real user data and simulated listener data. Real user data provides ground truth and context, while simulated listener data allows for comprehensive and controlled testing. This combined approach helps to create a more accurate and robust recommendation system.
Integrating “Robot Listener” Data into the AI System
The integration of “robot listener” data into Spotify’s AI system can be visualized through a flowchart.
[Imagine a flowchart here. The flowchart would begin with a box labeled “Generate Simulated Listener Profiles,” branching to “Expose Profiles to Recommendation Algorithm.” This would lead to “Collect Algorithm Output (Recommendations).” This then branches to “Analyze Algorithm Performance (Accuracy, Diversity, etc.).” The analysis feeds back into “Refine Algorithm and/or Simulated Listener Profiles,” creating a loop for continuous improvement. Finally, an arrow points from “Refine Algorithm” to “Deploy Improved Algorithm to Real Users.”]
This iterative process ensures the recommendation system continuously learns and adapts, becoming more effective at providing personalized music experiences.
Impact on Music Discovery and Consumption
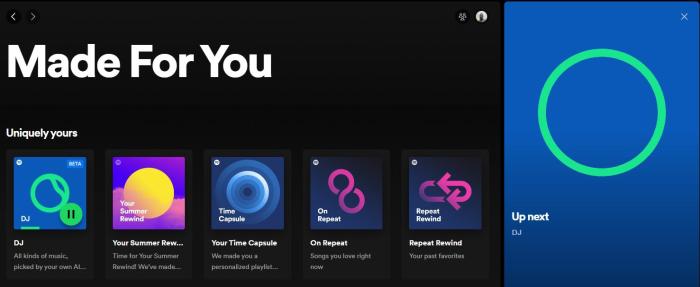
Source: co.zw
Spotify’s AI-powered music recommendation system dramatically reshapes how we discover and consume music, impacting both listeners and artists in profound ways. It’s a double-edged sword, offering incredible potential for personalized experiences but also raising concerns about bias and limited exposure.
AI-driven music discovery offers significant benefits for users and artists alike. For users, it’s about personalized playlists tailored to their tastes, leading to the discovery of new artists and genres they might never have encountered otherwise. For artists, it offers a powerful tool for reaching new audiences, bypassing the traditional gatekeepers of the music industry. Imagine a small indie band suddenly gaining thousands of listeners because their music perfectly aligns with the AI’s recommendation algorithm for a specific user demographic.
Benefits of AI-Driven Music Discovery, Spotify ai music robot listeners
AI’s impact on music consumption is multifaceted. The algorithm analyzes listening habits, identifying patterns and preferences to suggest similar artists and tracks. This personalized approach significantly enhances the user experience, making music discovery more efficient and enjoyable. For artists, increased visibility translates to more streams, higher profile, and ultimately, greater financial success. Consider the case of artists who’ve gained traction through viral TikTok trends—AI algorithms can play a similar role in amplifying reach, though often in a more targeted and nuanced way.
Influence on Music Trends and Popularity
Spotify’s AI doesn’t just react to trends; it actively shapes them. By promoting certain artists and genres through its recommendations, the algorithm can influence what becomes popular. This creates a feedback loop: the more a song is recommended, the more it’s listened to, further reinforcing its position in the charts. We’ve seen this with the rise of certain subgenres, where AI recommendations have played a significant role in their rapid expansion and mainstream acceptance. Conversely, artists who fail to align with the algorithm’s preferences may struggle to gain traction, regardless of their musical talent.
Potential Drawbacks and Biases of AI-Driven Music Recommendation Systems
The very personalization that makes AI recommendations so appealing can also lead to limitations. AI systems are trained on existing data, meaning they can perpetuate existing biases. If the data predominantly features artists from certain genres or demographics, the algorithm may underrepresent others, creating an echo chamber effect. This can inadvertently stifle the discovery of diverse musical styles and limit exposure for underrepresented artists. Moreover, relying solely on AI recommendations can lead to a lack of serendipitous discovery, the joy of stumbling upon something unexpected and amazing.
Comparison of User Experience with and Without AI-Driven Recommendations
Feature | With AI Recommendations | Without AI Recommendations |
---|---|---|
Discovery of New Music | Highly personalized and efficient; increased chance of finding music aligned with preferences. | Relies heavily on user initiative; potential for missing out on relevant music. |
Playlist Creation | Automated and tailored playlists; less time spent curating. | Requires manual selection and organization; more time-consuming. |
Exposure to Diverse Genres | Potentially limited due to filter bubbles; may reinforce existing preferences. | Greater potential for serendipitous discovery and exposure to diverse genres. |
Overall User Satisfaction | Generally high due to convenience and personalization, but potential for limited musical horizons. | Potentially lower due to effort required, but higher potential for musical exploration. |
Limitations of Music Discovery Due to Filter Bubbles
Spotify’s AI, while beneficial, can create filter bubbles. By constantly recommending music similar to what a user already listens to, the algorithm limits exposure to new and different genres or artists. This creates a self-reinforcing cycle, where users are only presented with music that confirms their existing preferences, potentially hindering musical growth and exploration. This effect is similar to how social media algorithms can reinforce existing political or social viewpoints, limiting exposure to alternative perspectives. The result is a less diverse and potentially less enriching musical landscape for the individual user.
Future Trends and Developments
Spotify’s AI-powered music recommendation engine is already a game-changer, but the future holds even more exciting possibilities. We’re on the cusp of a revolution in personalized audio experiences, driven by increasingly sophisticated algorithms and a deeper understanding of user preferences. This evolution will not only reshape how we discover and consume music but also significantly impact the music industry itself.
The coming years will see advancements in AI that go beyond simple “like” and “dislike” interactions. Imagine an AI that truly understands the nuances of your musical taste, predicting not just what you’ll like next, but *why* you’ll like it, based on subtle sonic patterns and emotional responses. This level of personalization will lead to a more intuitive and satisfying listening experience, moving beyond simple genre-based recommendations to a truly bespoke soundtrack for your life.
Spotify’s AI-powered robot listeners are constantly evolving, analyzing our listening habits with unsettling accuracy. It’s a whole different level of data mining compared to, say, the revamped subscription model for movie lovers detailed in this article on new moviepass features. Ultimately, both systems aim to predict our entertainment preferences, though one uses algorithms to curate playlists, and the other, movie tickets.
Enhanced User Experience Through Advanced AI
Future AI enhancements will focus on hyper-personalization. This means moving beyond simple genre recommendations to analyze subtle musical elements, contextual factors (like time of day or activity), and even your emotional state (inferred from listening patterns) to curate playlists. For instance, the AI might notice you listen to upbeat pop in the morning and mellow jazz in the evening and automatically adjust playlists accordingly. It could even learn to anticipate your mood based on your calendar events – crafting a focused playlist for a workout or a relaxing selection for a quiet evening. Imagine Spotify suggesting a playlist titled “Focus Flow: Instrumental Study Session” based on your current calendar entry showing a study session.
AI Integration with Social Features
Integrating AI with Spotify’s social features could create a dynamic and collaborative listening experience. Imagine AI suggesting collaborative playlists with friends based on shared listening habits, automatically generating playlists for parties or road trips based on the musical tastes of everyone involved. The AI could even analyze real-time social media trends and incorporate popular songs into personalized playlists, ensuring you’re always in the loop with what’s hot.
Impact of Increased Personalization on the Music Industry
Hyper-personalization will profoundly reshape the music industry. Independent artists will have unprecedented opportunities to reach targeted audiences, bypassing traditional gatekeepers. AI-powered analytics could help artists understand their fanbase better, allowing for more effective marketing and engagement. Conversely, major labels will need to adapt by leveraging AI to analyze trends and refine their A&R processes. This could lead to a more diverse musical landscape, with niche genres and independent artists gaining greater visibility.
A Glimpse into a Future Spotify Interface
Imagine a Spotify interface where your home screen isn’t just a list of playlists, but a dynamic, visually rich landscape reflecting your current mood and listening habits. A calming, pastel-colored interface might appear when you’re relaxing, while a vibrant, energetic design could accompany your workout playlist. Instead of scrolling through endless lists, you might interact with a visual representation of your music library, perhaps a constellation of stars, each representing a song or album, their brightness and color reflecting their relevance to your current mood or listening history. The AI could suggest new music by subtly shifting the constellation, highlighting promising new additions with shimmering effects. This immersive and interactive experience would make discovering new music feel less like a chore and more like a journey of sonic exploration.
AI-Driven Copyright and Royalty Distribution
AI can play a crucial role in addressing the complex issue of music copyright and royalty distribution. Advanced algorithms could more accurately identify and match copyrighted material, streamlining the process of royalty payments to artists and rights holders. This increased efficiency and transparency could help resolve long-standing disputes and ensure fairer compensation for creators. Imagine an AI system that automatically tracks the usage of each song across all platforms, instantly calculating and distributing royalties, eliminating much of the manual effort and potential for errors.
The User Experience of AI-Curated Playlists
Spotify’s AI-powered playlists have revolutionized how we discover and consume music. Unlike the painstakingly crafted playlists of old, these algorithmically generated selections offer a constantly evolving stream of tunes tailored to individual listening habits. But how does this user experience actually stack up against human-curated playlists, and what makes some AI playlists hit gold while others miss the mark entirely?
AI-generated playlists differ significantly from user-created ones in their scale and personalization. Human-curated playlists are often thematic, reflecting a specific mood, genre, or era. They’re limited by the curator’s knowledge and taste. In contrast, Spotify’s AI can analyze vast datasets of listening data – your own, and millions of others – to create personalized playlists that adapt and evolve based on your ongoing listening behavior. This means that an AI playlist can seamlessly blend familiar favorites with unexpected discoveries, offering a dynamic and ever-changing listening experience.
Comparison of Listening Experiences
The listening experience of AI-curated playlists is characterized by its surprising and personalized nature. While a human-curated playlist offers a cohesive and pre-determined listening journey, the AI version presents a more serendipitous path. You might encounter tracks you wouldn’t normally seek out, leading to exciting musical discoveries. Conversely, a human-curated playlist offers a curated experience, often reflecting a specific artistic vision or narrative, providing a more focused listening experience. The success of each hinges on different factors: the AI’s understanding of your tastes versus the curator’s artistic intent.
Factors Contributing to AI Playlist Success or Failure
The effectiveness of an AI-generated playlist is a complex interplay of factors. Accurate data is crucial; if the AI is fed incorrect or incomplete information about your listening preferences, the resulting playlist will likely be off the mark. The algorithm’s sophistication also plays a vital role; a more advanced algorithm can better understand nuances in musical taste and create more relevant playlists. Finally, the user’s own openness to new music is a key factor; if you’re resistant to venturing outside your comfort zone, even the best AI playlist might fall flat. A poorly designed algorithm, lacking sufficient data, or failing to account for the subtle shifts in your listening habits, will result in playlists that feel disjointed or uninspired. Conversely, a well-designed algorithm, paired with rich data, results in a playlist that feels deeply personal and insightful.
User Feedback on AI Playlist Quality and Effectiveness
User feedback on AI playlists is mixed, but reveals important insights into their strengths and weaknesses. Here’s a summary:
- Many users praise the personalized nature of AI playlists, highlighting the discovery of new artists and songs they would not have found otherwise.
- A common criticism revolves around the occasional inclusion of songs that don’t quite fit the overall mood or style of the playlist.
- Some users find that the AI’s suggestions become repetitive over time, lacking sufficient variety.
- Others express frustration with the lack of control over the playlist’s content, wishing for more options to customize or refine the selections.
- Positive feedback often centers on the convenience and effortless nature of having a constantly updated playlist tailored to individual tastes.
Emotional Response to AI vs. Human-Curated Playlists
The emotional response elicited by AI-curated playlists often differs from that of human-curated ones. Human-curated playlists can evoke a strong sense of nostalgia, shared experience, or artistic appreciation. They often tell a story or reflect a specific mood in a coherent way. AI-generated playlists, on the other hand, tend to foster a sense of surprise and discovery. The unexpected nature of the track selection can lead to feelings of excitement and intrigue. However, the lack of a unifying theme might result in a less emotionally resonant experience compared to a thoughtfully curated playlist that evokes a specific mood or memory. The emotional impact, therefore, depends heavily on the individual listener and their openness to the unpredictable nature of algorithmic curation.
Final Wrap-Up: Spotify Ai Music Robot Listeners
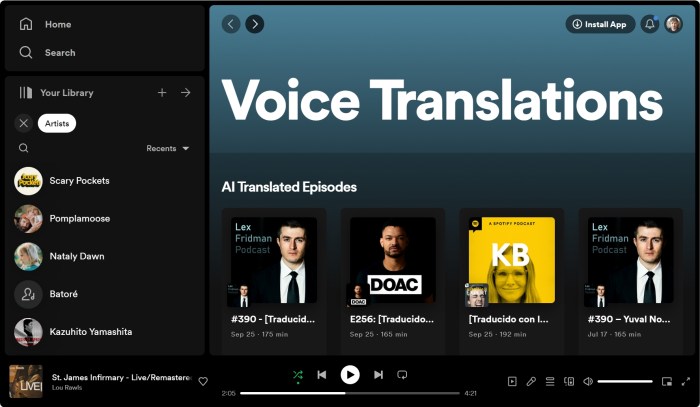
Source: winbuzzer.com
From the intricate workings of Spotify’s AI recommendation system to the ethical considerations of using simulated listeners, the journey into the world of algorithmic music curation has been a revealing one. The power of AI to personalize our musical journeys is undeniable, but so are the potential pitfalls. As AI continues to evolve, ensuring fairness, transparency, and a diverse musical landscape will be crucial. The future of music discovery, it seems, rests in the delicate balance between human creativity and artificial intelligence.