Unfair automated hiring systems are everywhere ftc – Unfair automated hiring systems are everywhere, FTC, and they’re quietly screwing over job seekers. These algorithms, meant to streamline the hiring process, are often biased, perpetuating existing inequalities and leaving qualified candidates overlooked. We’re diving deep into how these systems discriminate, the FTC’s role in stopping them, and what you need to know to protect yourself.
From subtle biases embedded in the data to outright discriminatory algorithms, the problem is widespread. This isn’t just about fairness; it’s about economic opportunity and social justice. We’ll explore the different types of bias, the devastating impact on marginalized communities, and what steps can be taken to create a more equitable hiring landscape. Get ready to unpack the messy reality of AI in hiring.
Types of Bias in Automated Hiring Systems
The seemingly objective world of algorithms is, unfortunately, not immune to human biases. Automated hiring systems, designed to streamline the recruitment process, can inadvertently perpetuate and even amplify existing societal inequalities. These systems, trained on historical data, often reflect the biases present in that data, leading to discriminatory outcomes for certain groups. Understanding the different types of bias embedded within these systems is crucial to mitigating their harmful effects and building a fairer hiring process.
Bias in automated hiring systems manifests in various ways, stemming from both the data used to train the algorithms and the algorithms themselves. This results in unfair and discriminatory practices, often impacting underrepresented groups disproportionately. The consequences can be far-reaching, hindering career advancement and perpetuating economic inequality.
The FTC’s cracking down on unfair automated hiring systems is long overdue; these algorithms often perpetuate existing biases. Think about it – the same tech powering cool stuff like dall e 3 open ai chat gpt can also be used to unfairly screen job applicants. This highlights the urgent need for ethical AI development and responsible deployment across all sectors, especially in hiring practices.
Data Bias in Automated Hiring Systems
Data bias occurs when the data used to train an algorithm is not representative of the broader population. This can stem from historical hiring practices that favored certain demographics, leading to skewed datasets that reflect those biases. For instance, if a dataset predominantly contains information on white male applicants who have been hired in the past, the algorithm may learn to favor similar candidates, disadvantaging women and people of color. This type of bias is often unintentional but has significant consequences. The algorithm isn’t inherently prejudiced; it simply reflects the prejudices embedded within the data it was trained on.
Algorithmic Bias in Automated Hiring Systems
Even with a perfectly representative dataset, algorithmic bias can still occur. This arises from flaws in the algorithm’s design or implementation. For example, an algorithm might disproportionately weigh certain factors, such as years of experience at a specific company, which could inadvertently disadvantage candidates from less privileged backgrounds who may have less access to such opportunities. Another example is the use of proxies: the algorithm might use seemingly neutral factors like zip code, which can indirectly correlate with race and socioeconomic status, leading to discriminatory outcomes.
Examples of Biased Algorithms and Tools
Several instances of biased algorithms in hiring have been documented. One example involves resume screening tools that penalized applicants with names commonly associated with certain racial or ethnic groups. Another case involved an algorithm used for candidate ranking that systematically downgraded applications from women, even when controlling for other relevant factors. These instances highlight the real-world consequences of biased algorithms, impacting individuals’ career prospects and perpetuating systemic inequalities. The impact can range from missed opportunities to the reinforcement of existing biases in the workplace.
Comparison of Bias Types and Effects, Unfair automated hiring systems are everywhere ftc
Bias Type | Description | Example | Impact |
---|---|---|---|
Data Bias | Bias stemming from non-representative historical data used to train the algorithm. | A hiring algorithm trained on data primarily reflecting white male hires may favor similar candidates, disadvantaging women and minorities. | Discriminatory outcomes against underrepresented groups; reinforcement of existing inequalities. |
Algorithmic Bias | Bias resulting from flaws in the algorithm’s design or implementation, even with representative data. | An algorithm prioritizing years of experience at a specific company might disadvantage candidates from less privileged backgrounds with fewer such opportunities. | Unfair disadvantage to certain groups based on factors indirectly related to merit; perpetuation of systemic inequalities. |
Proxy Bias | Bias introduced through the use of seemingly neutral variables that correlate with protected characteristics. | Using zip code as a predictor, inadvertently disadvantaging candidates from lower-income neighborhoods often associated with specific racial or ethnic groups. | Indirect discrimination; masking of true biases within the algorithm. |
Confirmation Bias | The algorithm reinforces existing beliefs or prejudices by prioritizing information confirming those biases. | An algorithm that prioritizes candidates from elite universities, reflecting a pre-existing bias towards those institutions. | Limited diversity of thought and perspectives; perpetuation of elitism. |
Impact of Unfair Automated Hiring Systems on Job Seekers
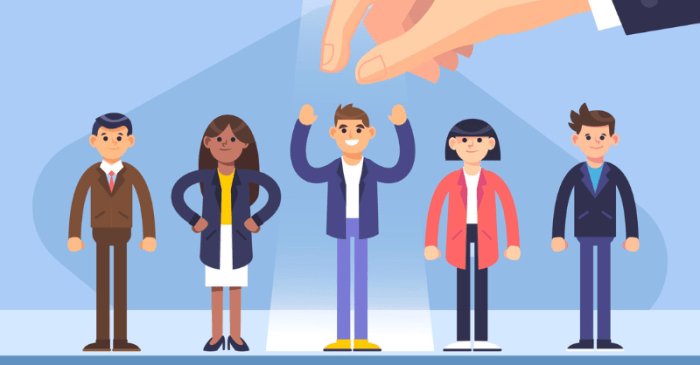
Source: skillrobo.com
The rise of automated hiring systems, while promising efficiency, has cast a long shadow on job seekers, particularly those from marginalized communities. These systems, often trained on biased data, perpetuate and amplify existing inequalities, leading to significant economic and social consequences for many. The impact extends beyond simple rejection; it creates a cycle of disadvantage that limits opportunities and reinforces systemic biases.
The economic and social repercussions of unfair automated hiring systems are far-reaching and deeply damaging for marginalized groups. For example, algorithms trained on historical hiring data may inadvertently discriminate against women or people of color, who have historically been underrepresented in certain industries. This leads to lower employment rates, reduced earning potential, and a widening wealth gap. The social impact includes decreased self-esteem, feelings of exclusion, and a loss of faith in fair and equitable employment practices. This can further exacerbate existing societal inequalities and create a sense of hopelessness among affected individuals.
Examples of Perpetuated Inequalities
Algorithmic bias in hiring can manifest in several ways. Resume screening tools, for instance, might penalize candidates with unconventional names or those who attended less prestigious universities, disproportionately affecting minority groups and individuals from lower socioeconomic backgrounds. Similarly, video interviewing software might inadvertently favor candidates with certain communication styles or physical appearances, disadvantaging those who don’t fit a specific, often unconsciously biased, ideal. These systems, therefore, aren’t simply neutral tools; they actively reinforce pre-existing biases embedded within the data they’re trained on. This can lead to a situation where qualified candidates from marginalized groups are systematically overlooked, perpetuating the cycle of inequality within the workforce.
Comparison of Job Seeking Methods
Job seekers using online application methods often face a higher likelihood of encountering biased automated systems. The impersonal nature of online applications, coupled with the algorithmic screening processes, can lead to a significant disadvantage for candidates who may excel in in-person interactions but lack the specific s or formatting favored by the automated system. In contrast, those who rely on in-person networking and referrals often bypass these initial automated filters, highlighting the disparity in access to opportunities based on the method of job seeking. This disparity creates an uneven playing field where some candidates have an inherent advantage simply because of their access to networks and opportunities that circumvent biased algorithms.
Case Study: The Algorithmic Exclusion of Aspiring Teachers
Consider a hypothetical scenario involving a large urban school district using an automated system to screen teacher applications. The system, trained on historical hiring data, may unintentionally prioritize candidates with certain backgrounds and experiences, potentially overlooking highly qualified individuals from underrepresented minority groups. For example, the algorithm might favor candidates with specific types of teaching certifications or from certain universities, effectively excluding equally or more qualified teachers from diverse backgrounds who may have pursued alternative pathways to certification or attended less prestigious institutions. This results in a less diverse teaching staff, potentially impacting the educational experience of students from diverse backgrounds and failing to provide role models for those communities. This is not merely a hypothetical; similar biases have been documented in various sectors, demonstrating the real-world consequences of these systems.
Mitigating Bias in Automated Hiring Systems: Unfair Automated Hiring Systems Are Everywhere Ftc
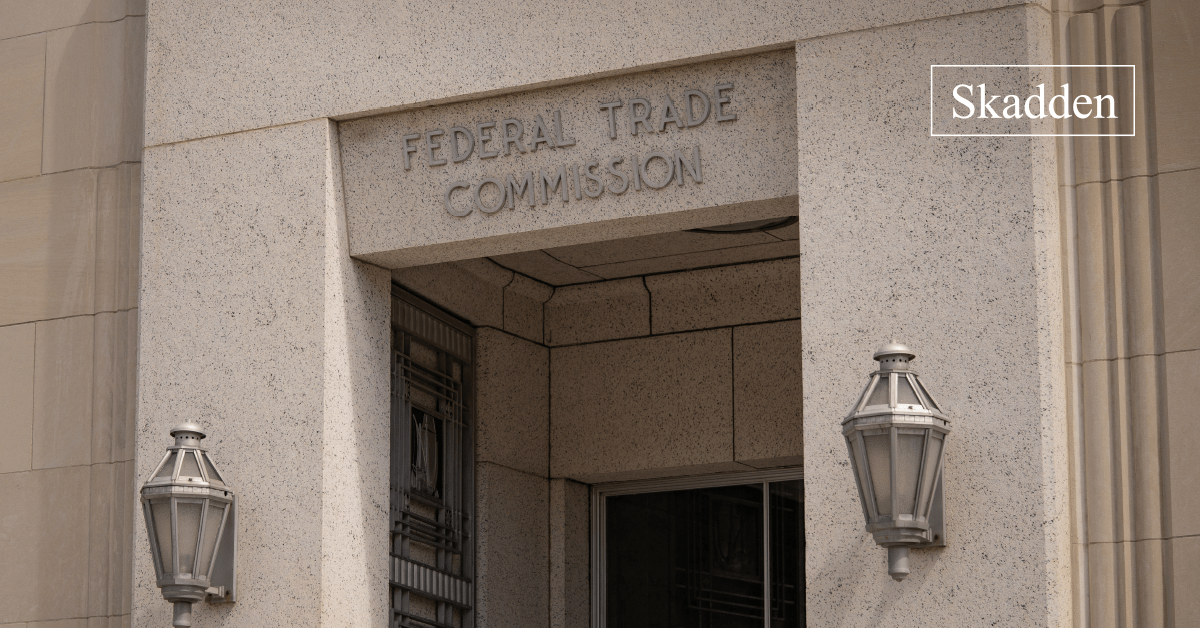
Source: skadden.com
The chilling reality is that biased algorithms are quietly shaping our job markets. But the good news is, we can actively fight back. Mitigating bias in automated hiring systems isn’t just about ethical responsibility; it’s about building a fairer, more efficient, and ultimately more successful workforce. This involves a multi-pronged approach, from careful data collection to rigorous testing and ongoing audits.
Building fair and unbiased automated hiring systems requires a proactive and multifaceted strategy. It’s not a one-time fix but an ongoing commitment to transparency and accountability. Ignoring this crucial aspect can lead to significant legal and reputational risks, not to mention the perpetuation of systemic inequalities.
Diverse Datasets and Robust Testing Methodologies
The foundation of any unbiased system lies in the data it’s trained on. A dataset reflecting the diversity of the applicant pool is paramount. This means actively seeking out and including data from underrepresented groups, ensuring a representative sample across gender, race, ethnicity, age, socioeconomic background, and other relevant demographic factors. A skewed dataset will inevitably lead to a skewed outcome. Further, rigorous testing is crucial. This goes beyond simply checking for accuracy; it involves actively testing for bias using various statistical methods and simulations to identify and correct any discriminatory patterns. For example, if an algorithm consistently favors candidates from specific universities or with certain s in their resumes, this indicates potential bias needing immediate attention. Think of it as a rigorous quality control process, but for fairness.
Auditing Existing Automated Hiring Systems for Bias
Existing systems aren’t immune to bias; in fact, they may already be perpetuating it. Regular audits are vital. This involves analyzing the system’s decision-making process to identify any patterns that disproportionately favor or disadvantage specific groups. Techniques like fairness-aware machine learning algorithms and statistical methods such as disparate impact analysis can be employed to pinpoint these biases. For instance, if the system consistently rejects applications from candidates with certain names, even when their qualifications are comparable to those of accepted candidates, it’s a clear indication of bias. The goal of these audits isn’t just to find problems, but to understand their root causes and implement effective solutions.
Checklist for Ensuring Fairness and Transparency in Hiring
Companies need a concrete plan to address bias. This checklist provides a structured approach:
- Data Assessment: Thoroughly review your existing data for potential biases. Identify any gaps in representation and create a plan to address them.
- Algorithm Selection: Choose algorithms known for their fairness and transparency. Avoid “black box” systems where the decision-making process is opaque.
- Bias Mitigation Techniques: Implement techniques such as pre-processing, in-processing, and post-processing methods to actively mitigate bias in the data and algorithms.
- Regular Audits and Monitoring: Conduct regular audits to detect and correct biases over time. Establish clear metrics to track fairness and transparency.
- Human Oversight: Maintain human oversight in the hiring process. Automated systems should support, not replace, human judgment.
- Transparency and Explainability: Ensure the system’s decision-making process is transparent and explainable to both candidates and stakeholders.
- Employee Training: Educate hiring managers and HR personnel on the potential for bias in automated systems and best practices for fair hiring.
- Feedback Mechanisms: Establish mechanisms for candidates to provide feedback on their experiences with the automated hiring system.
Implementing these steps is not merely a compliance exercise; it’s a strategic investment in a more equitable and effective workforce. By prioritizing fairness and transparency, companies can cultivate a more inclusive environment, attracting and retaining top talent from diverse backgrounds.
The Role of Transparency and Accountability
The rise of automated hiring systems promises efficiency, but their opaque nature raises serious concerns about fairness and equal opportunity. Transparency and accountability are not just buzzwords; they’re fundamental requirements to ensure these systems don’t perpetuate existing biases and inadvertently discriminate against qualified candidates. Without them, we risk creating a technologically advanced system that’s fundamentally unjust.
Algorithmic decision-making in hiring must be transparent to build trust and allow for scrutiny. This means companies need to be open about the data used to train their algorithms, the specific algorithms themselves (to the extent commercially viable), and the factors influencing their hiring decisions. This transparency allows for independent audits and enables job seekers to understand why they were or weren’t selected, fostering a sense of fairness and promoting accountability. Lack of transparency fuels distrust and reinforces the perception of algorithmic bias, hindering the acceptance and effective use of these systems.
Explaining Automated Hiring Decisions to Applicants
Providing clear and understandable explanations to applicants is crucial for building trust and addressing concerns about bias. Simply stating “You were not selected” is insufficient. A more effective approach involves providing a summary of the applicant’s strengths and weaknesses based on the algorithm’s assessment, while acknowledging the limitations of the system. For example, an explanation could highlight that the applicant’s skills matched well with certain aspects of the job description but lacked experience in a specific area deemed crucial by the algorithm. This approach allows applicants to understand the decision-making process and identify areas for improvement, rather than leaving them feeling unfairly rejected. Furthermore, offering this level of detail allows for the identification of potential biases within the system itself. A consistent pattern of unfairly penalizing candidates for specific characteristics (e.g., certain schools, gaps in employment) could indicate a flaw in the algorithm’s design.
A Framework for Reporting and Addressing Complaints
A robust framework for reporting and addressing complaints about biased automated hiring systems is essential. This framework should include clearly defined channels for submitting complaints, a process for investigating those complaints, and a mechanism for resolving disputes. The process should be accessible, transparent, and impartial, ensuring that complaints are taken seriously and addressed promptly. The investigation should involve an independent review of the algorithm’s decision-making process, the data used, and the applicant’s qualifications. This review should determine whether the system operated as intended and whether any biases were present. If bias is found, corrective actions should be taken, potentially including retraining the algorithm, modifying its parameters, or even replacing the system altogether. This framework needs to include provisions for redress, such as offering applicants a second chance or compensating them for any damages suffered due to the discriminatory practices. Examples of successful frameworks could draw from existing regulatory mechanisms for employment discrimination, adapting them to the unique challenges posed by automated systems. A dedicated team or external auditor could oversee this process, ensuring its impartiality and effectiveness.
Future Regulatory Landscape
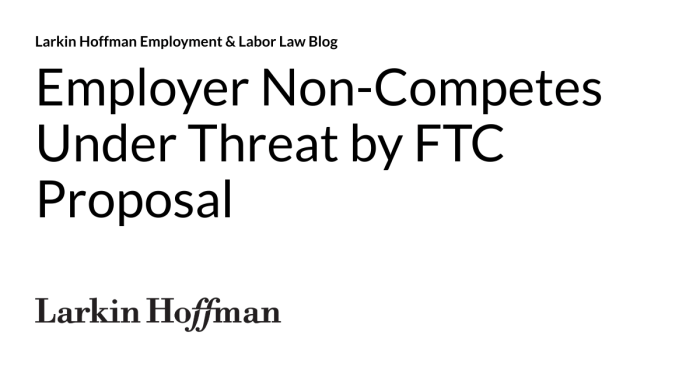
Source: bannerbear.com
The increasing prevalence of biased automated hiring systems is pushing governments worldwide to consider stricter regulations. The potential for these systems to perpetuate and amplify existing societal inequalities demands a proactive and comprehensive regulatory response. This involves a complex balancing act: protecting job seekers from discrimination while fostering innovation in the tech sector.
The future regulatory landscape for AI in hiring is likely to be a patchwork of national and international initiatives, each with its own nuances. However, several common themes are emerging, driven by the need for fairness, transparency, and accountability.
Potential Future Regulations
Several regulatory approaches are being explored globally. These range from outright bans on specific AI hiring tools deemed discriminatory to mandates requiring rigorous audits and impact assessments before deployment. Some jurisdictions are focusing on data privacy regulations, recognizing that biased datasets are the root of many problems. Others are emphasizing the need for algorithmic transparency, giving job applicants the right to understand how AI systems evaluate their applications. For example, the EU’s AI Act proposes a risk-based approach, classifying AI systems based on their potential harm and imposing stricter requirements on high-risk applications, which would certainly include those used in hiring. The US, in contrast, is pursuing a more piecemeal approach, with various states enacting their own specific regulations. California, for instance, has legislation requiring employers to conduct impact assessments for automated decision-making systems.
Comparative Analysis of Regulatory Approaches
A comparison of different regulatory approaches reveals a spectrum of methods. The EU’s AI Act, with its risk-based classification, represents a comprehensive and arguably stricter approach than the more fragmented approach of the US, which relies heavily on existing anti-discrimination laws and emerging state-level regulations. Canada’s approach is also evolving, with a focus on algorithmic transparency and accountability, similar to but perhaps less prescriptive than the EU’s. This diversity highlights the challenges in establishing a universally accepted regulatory framework, reflecting the varying cultural, legal, and technological contexts.
Impact of New Regulations on Businesses and Job Seekers
New regulations will undoubtedly impact businesses, requiring them to invest in auditing, testing, and potentially redesigning their AI hiring systems. This could increase compliance costs and necessitate changes to internal processes. However, it could also lead to more robust and fairer hiring practices, ultimately benefiting both businesses and job seekers. For job seekers, clearer regulations could lead to greater transparency and accountability in the hiring process, potentially reducing instances of unfair or discriminatory practices. They might gain better access to information about how AI is used in hiring decisions, enabling them to challenge potentially biased outcomes. However, over-regulation could also lead to unintended consequences, such as limiting the use of beneficial AI tools or increasing processing times for applications.
Visual Representation of the Future Regulatory Landscape
Imagine a global map. Different countries are represented by colored regions, each color representing a distinct regulatory approach: a deep blue for the comprehensive, risk-based approach (like the EU); a lighter blue for a more fragmented, state-level approach (like the US); a green for a focus on transparency and accountability (like Canada). Overlapping regions represent countries adopting hybrid approaches, incorporating elements from multiple regulatory styles. The intensity of the color could represent the strictness of the regulations within that region. Arrows between regions illustrate the influence and cross-pollination of ideas and regulatory frameworks across borders, depicting the evolving and dynamic nature of the global regulatory landscape for AI in hiring.
Concluding Remarks
The fight against unfair automated hiring systems is far from over, but understanding the issues – the biased algorithms, the FTC’s potential role, and the impact on job seekers – is the first step towards real change. We need transparency, accountability, and a commitment to developing ethical AI in hiring. The future of work depends on it. Let’s demand better.