Remotasks AI expert data labor isn’t just about clicking buttons; it’s the unseen engine powering the AI revolution. Think of it as the secret sauce, the tireless team meticulously labeling the data that fuels self-driving cars, facial recognition software, and even your favorite language translation app. This intricate dance between human expertise and artificial intelligence is shaping the future, one carefully annotated dataset at a time. We’ll dive deep into the world of Remotasks, exploring the roles of AI experts, the challenges they face, and the massive impact their work has on the tech landscape.
From understanding the platform’s core functionalities to navigating the diverse data annotation tasks, we’ll uncover the skills needed to become a Remotasks AI expert. We’ll compare the roles of human annotators and AI, examining the unique contributions of each. We’ll also explore the ethical considerations and future trends that are reshaping this exciting field. Get ready for a behind-the-scenes look at how your digital world is built, one data point at a time.
Remotasks Platform Overview
Remotasks is a crowdsourcing platform that connects businesses with a global workforce to perform data annotation tasks crucial for training artificial intelligence models. It’s essentially a digital factory for AI, providing the human-in-the-loop element needed to refine and improve machine learning algorithms. Think of it as a bridge between human expertise and the power of AI.
Remotasks core functionalities revolve around task creation, worker management, and quality control. Businesses can upload their data and define specific annotation instructions, while Remotasks manages the distribution of these tasks to its global network of workers. The platform then employs various quality control mechanisms to ensure accuracy and consistency, ultimately delivering high-quality annotated data for AI training.
Data Annotation Tasks on Remotasks
The types of data annotation tasks available on Remotasks are incredibly diverse, reflecting the broad applications of AI. Workers might be involved in image annotation (bounding boxes, semantic segmentation, polygon annotation), text annotation (sentiment analysis, named entity recognition, text categorization), audio annotation (transcription, speaker diarization, sound event detection), and video annotation (object tracking, action recognition, event detection). The complexity and specificity of these tasks vary greatly, catering to different AI model development needs. For example, one task might involve simply drawing boxes around cars in images, while another might require nuanced labeling of emotions expressed in speech.
The Role of AI in the Remotasks Workflow
AI plays a significant, albeit supporting, role within the Remotasks workflow. While human annotators remain the core of the process, AI assists in several key areas. Firstly, AI-powered tools can automate parts of the annotation process, speeding up workflows and reducing the workload on human annotators. Secondly, AI helps in quality control, flagging potentially problematic annotations for review by supervisors. Thirdly, AI algorithms can help in the initial organization and pre-processing of the data before it’s distributed to annotators. This intelligent automation ensures efficiency and consistency throughout the entire data annotation lifecycle. Imagine an AI pre-sorting images based on content, making it easier for human annotators to focus on their specific task.
Completing a Simple Remotasks Task: A Step-by-Step Guide
Let’s say a new user is tasked with labeling images containing cats and dogs. First, the user would log in to the Remotasks platform and access the assigned task. The platform will provide clear instructions, including examples of correctly labeled images. The user would then proceed to view each image individually. Using the provided tools, they would draw bounding boxes around each cat and dog, assigning the appropriate labels (“cat” or “dog”). After completing the labeling for an image, they would submit it for review. The platform will then provide feedback on the accuracy of the labeling, helping the user improve their performance over time. This iterative process ensures high-quality annotations are consistently produced.
AI Expert Involvement in Remotasks
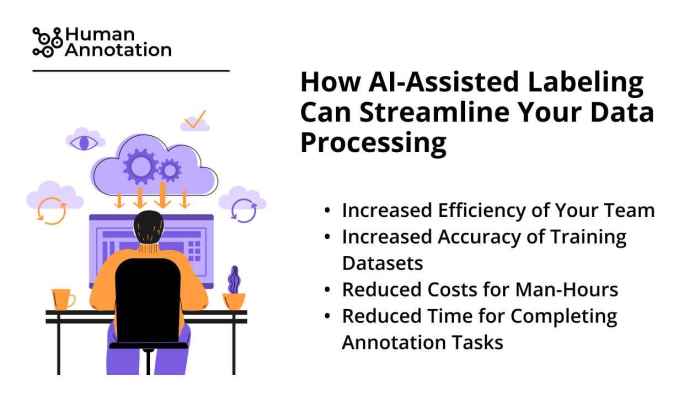
Source: humanannotation.com
Remotasks isn’t just about crowdsourcing data; it’s about leveraging the power of human intelligence combined with sophisticated AI. AI experts are crucial to the platform’s success, playing a vital role in ensuring the quality, accuracy, and efficiency of data annotation projects. They’re the brains behind the operation, guiding the process and refining the algorithms that ultimately power AI advancements.
AI experts working with Remotasks are far more than just data labelers. They act as quality control specialists, algorithm trainers, and project strategists, ensuring the data produced meets the stringent requirements of clients developing cutting-edge AI applications. Their expertise bridges the gap between raw data and actionable insights.
Required Skills and Qualifications for AI Experts
Success on the Remotasks platform as an AI expert requires a blend of technical skills and soft skills. Crucially, a strong understanding of machine learning principles and algorithms is essential. This isn’t just theoretical knowledge; it translates into practical experience with various annotation tools and techniques. Proficiency in programming languages like Python is almost always a necessity, along with familiarity with popular machine learning libraries such as TensorFlow and PyTorch. Beyond technical skills, strong problem-solving abilities, attention to detail, and the ability to communicate effectively with both technical and non-technical teams are vital for success. Experience with specific AI models, such as object detection, image classification, or natural language processing, will significantly enhance an expert’s value.
Roles of AI Experts in Data Annotation Projects
AI experts on Remotasks fulfill a variety of crucial roles within data annotation projects. They often act as project managers, overseeing the entire annotation workflow, defining quality standards, and ensuring the timely completion of tasks. They design and implement quality control procedures, identifying and resolving inconsistencies in the annotated data. Furthermore, AI experts play a pivotal role in algorithm training, using the annotated data to refine and improve the performance of machine learning models. This includes designing training datasets, evaluating model performance, and iteratively improving the models’ accuracy and efficiency. Finally, they may also be involved in developing and deploying new annotation tools and workflows to optimize the annotation process.
Comparison of AI Expert and Human Annotator Tasks
While both AI experts and human annotators contribute to data annotation on Remotasks, their roles differ significantly. Human annotators primarily focus on the meticulous task of labeling data according to predefined guidelines. They are the hands-on workers, providing the raw, labeled data. AI experts, however, take a more strategic and oversight role. They design the annotation guidelines, train and evaluate the algorithms that process the human-annotated data, and implement quality control measures. Essentially, human annotators provide the data, while AI experts ensure its quality, accuracy, and utility in training AI models. This synergistic relationship is key to the platform’s effectiveness.
Challenges and Rewards of Being an AI Expert on Remotasks
Working as an AI expert on Remotasks presents unique challenges and rewards. One of the primary challenges is the constant need to adapt to new technologies and project requirements. The field of AI is rapidly evolving, demanding continuous learning and skill development. Maintaining data quality and consistency across large-scale projects can also be demanding. However, the rewards are equally compelling. AI experts gain valuable experience working on diverse and challenging projects, contributing directly to the advancement of AI technologies. The flexible work arrangements and the opportunity to collaborate with a global community of experts also make it an attractive career path for many. Furthermore, the direct impact on the development of cutting-edge AI systems offers a significant sense of professional fulfillment.
Data Labor and its Impact
Data labeling might sound like a techie term, but it’s the unsung hero powering the AI revolution. Think of it as teaching a computer to see, hear, and understand the world – all thanks to the meticulous work of human data labelers on platforms like Remotasks. This process is crucial for developing accurate and reliable AI systems, and its impact is far-reaching and increasingly vital in our increasingly data-driven world.
The work done by Remotasks’ data labelers is the backbone of many AI advancements. Without this human-in-the-loop process, AI would remain a theoretical concept, unable to translate raw data into the actionable insights that drive innovation across various sectors. Let’s dive into the specifics of this essential work.
Types of Data Labeled by Remotasks Workers
Remotasks workers handle a diverse range of data labeling tasks. They might annotate images by identifying objects, classifying scenes, or drawing bounding boxes around specific items. For audio data, tasks include transcribing speech, identifying different speakers, or labeling audio events like laughter or applause. Text data labeling involves tasks such as sentiment analysis (determining the emotional tone of text), named entity recognition (identifying people, places, and organizations), or categorizing text into different topics. The complexity varies greatly depending on the project’s needs, ranging from simple binary classifications to highly nuanced semantic annotations. For example, one task might involve simply identifying whether an image contains a cat, while another might require detailed annotation of the cat’s breed, pose, and surrounding environment.
Industries Benefiting from Remotasks Data Labeling Services
The applications of Remotasks’ data labeling services span numerous industries. Self-driving car companies rely heavily on labeled image and sensor data to train their AI systems to navigate roads safely. Healthcare utilizes labeled medical images for diagnosing diseases and developing AI-powered diagnostic tools. Retail businesses leverage labeled product images and customer reviews to improve their search algorithms and recommendation systems. The financial sector employs labeled transaction data to detect fraud and prevent money laundering. Even the entertainment industry uses labeled data to improve video game AI and create more realistic virtual environments. Essentially, any industry utilizing AI benefits from the high-quality data labeling provided by Remotasks.
Stages of a Typical Data Labeling Project on Remotasks
A typical data labeling project on Remotasks usually follows several key stages. First, the client provides the raw data – this could be images, audio files, text documents, or any other type of data needing annotation. Then, Remotasks designs the task instructions and quality control procedures specific to the client’s needs. Next, the data is distributed to qualified workers who perform the labeling tasks. Throughout the process, quality control measures are implemented to ensure accuracy and consistency. Finally, the labeled data is delivered back to the client, ready for use in AI model training. This iterative process, with its built-in quality checks, guarantees the delivery of high-quality, reliable data.
Hypothetical Workflow: AI and Human Data Labelers
Imagine a project involving the classification of satellite images to identify areas affected by deforestation. First, the AI system, trained on a smaller, pre-labeled dataset, automatically classifies the images. However, the AI’s classifications are not perfect and are prone to errors. Human labelers then review a subset of the AI’s classifications, correcting any mistakes and providing feedback on the AI’s performance. This feedback is then used to retrain the AI model, improving its accuracy over time. This collaborative workflow, leveraging both the speed of AI and the precision of human judgment, represents an efficient and effective approach to data labeling, resulting in significantly improved AI model performance. The AI handles the bulk of the initial work, while human expertise focuses on the more challenging or ambiguous cases, ensuring a high standard of accuracy in the final labeled dataset.
Quality Control and Assurance
Data quality is the lifeblood of any AI project, and at Remotasks, we take it seriously. Garbage in, garbage out – that’s the mantra we live by. Our commitment to delivering high-quality data involves a multi-layered approach, combining human expertise with the power of AI to ensure accuracy and consistency across all our projects. This rigorous process is what sets us apart and allows us to provide our clients with the reliable data they need to build truly effective AI models.
Remotasks employs a robust system of quality control measures to guarantee the accuracy and reliability of the data annotated by our global workforce. This system leverages both human review and automated AI-powered checks, ensuring a high level of precision and consistency. The process is designed to identify and correct errors early, preventing flawed data from impacting downstream applications. Our goal is not just to meet client expectations but to exceed them, delivering data that’s not only accurate but also efficiently collected and ready for immediate use.
Quality Control Methods at Remotasks
Remotasks utilizes several strategies to maintain data quality. These include multiple annotations per task, quality checks by experienced reviewers, and automated AI-based validation. Each method plays a crucial role in ensuring the overall accuracy and consistency of the data. The combination of these approaches minimizes human error and provides a robust safety net against data inconsistencies.
Quality Control Method | Description | Benefits | Limitations |
---|---|---|---|
Multiple Annotations | Each task is completed by multiple annotators, allowing for comparison and identification of discrepancies. | Increased accuracy and reliability through consensus. Highlights inconsistencies for targeted review. | Increased cost and time compared to single annotation. Requires robust conflict resolution mechanisms. |
Expert Review | Experienced annotators review a sample of completed tasks to identify and correct errors. | High accuracy for complex or ambiguous tasks. Provides valuable feedback for improving annotation guidelines. | Can be time-consuming and expensive, particularly for large datasets. Relies on the expertise and consistency of the reviewers. |
AI-Powered Validation | Machine learning models are used to automatically identify and flag potential errors in the annotations. | Efficient and scalable. Can identify subtle errors that might be missed by human reviewers. | Relies on the accuracy of the underlying AI model. May require human intervention for complex or ambiguous cases. |
Automated Consistency Checks | Algorithms compare annotations across multiple annotators to detect inconsistencies and flag them for review. | Identifies discrepancies early in the process. Allows for timely correction and prevents propagation of errors. | Requires well-defined annotation guidelines and clear criteria for consistency. May need adjustment based on project specifics. |
AI’s Role in Quality Control
AI plays a vital role in ensuring data quality at Remotasks. It goes beyond simply flagging potential errors; AI algorithms are trained to identify patterns and anomalies in the data, helping to predict and prevent inconsistencies before they occur. For example, AI can detect if an annotator is consistently deviating from established guidelines, prompting further review and potentially retraining. This proactive approach minimizes errors and improves the overall efficiency of the quality control process. Moreover, AI-driven tools can automate repetitive tasks, freeing up human reviewers to focus on more complex and nuanced issues.
Addressing Data Annotation Inconsistencies
Inconsistencies in data annotation are inevitable, but Remotasks has developed effective strategies to mitigate their impact. Our system uses a combination of automated detection and human review to identify and resolve discrepancies. When inconsistencies are detected, the system automatically flags the affected tasks for review by experienced annotators. These experts analyze the conflicting annotations, determine the correct labeling, and provide feedback to improve annotation guidelines and potentially retrain annotators to prevent similar issues in the future. This iterative process continuously refines the annotation process, leading to improved accuracy and consistency over time. Furthermore, clear and comprehensive annotation guidelines, coupled with regular training and feedback sessions for annotators, significantly reduce the likelihood of inconsistencies arising in the first place.
Future Trends and Implications: Remotasks Ai Expert Data Labor
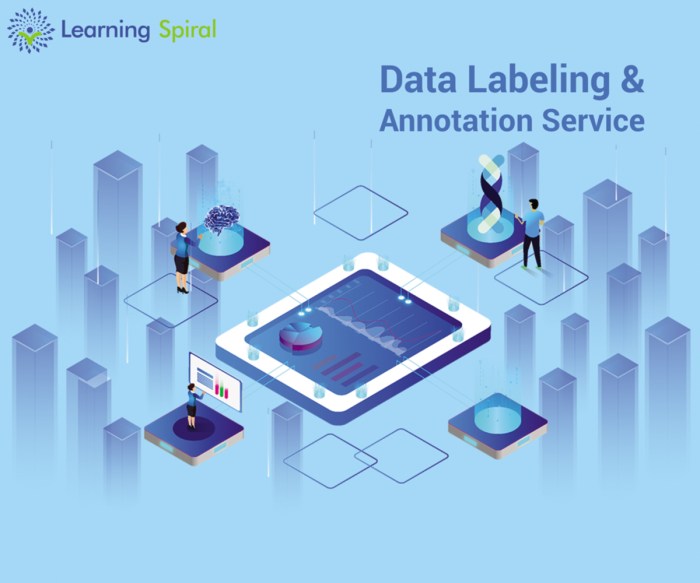
Source: learningspiral.ai
Remotasks’ AI expert data labor fuels advancements in various fields, but even the most advanced tech can’t escape the swirling narratives around us. The recent hype about 15 minute cities conspiracy climate denier shows how easily misinformation spreads, impacting public perception of even seemingly unrelated technologies like AI. Ultimately, the quality of data used to train AI, provided by platforms like Remotasks, remains crucial for responsible technological progress.
The world of AI is rapidly evolving, and with it, the demand for high-quality training data is exploding. Remotasks, as a key player in data annotation, is poised to ride this wave, but its future trajectory will be shaped by several significant trends and their associated implications. The platform’s success hinges on adapting to these changes, embracing innovation, and navigating the ethical complexities that arise.
The future of Remotasks’ data annotation services extends far beyond its current capabilities. We can expect to see a significant expansion into new domains, driven by the increasing sophistication of AI applications. This will involve not only handling larger datasets but also working with increasingly complex data types and annotation tasks.
Potential Applications of Remotasks’ Data Annotation Services
Remotasks’ expertise in data annotation can be leveraged across a vast spectrum of emerging AI applications. For example, the platform could play a crucial role in training AI models for autonomous vehicles, enabling them to better perceive and navigate complex environments. Another key area is medical imaging analysis, where accurate annotation of medical scans is vital for developing diagnostic tools and personalized treatments. Finally, advancements in natural language processing (NLP) will require increasingly nuanced data annotation, a service Remotasks is well-positioned to provide. The potential applications are virtually limitless, extending to areas like robotics, smart cities, and environmental monitoring.
Advancements in AI Transforming the Remotasks Platform
AI itself is set to revolutionize the Remotasks platform. Imagine automated quality control systems that use AI to identify and flag inconsistencies in annotations, reducing the need for manual review and significantly speeding up the process. Furthermore, AI-powered tools could assist annotators by suggesting labels or highlighting areas requiring attention, increasing both efficiency and accuracy. This could involve the implementation of machine learning algorithms that learn from previous annotations, predicting and pre-filling labels for subsequent tasks. This automated assistance could also personalize the annotation workflow, adapting to individual annotator skill levels and preferences.
Ethical Considerations Surrounding AI-Assisted Data Labeling
As AI takes on a more significant role in data labeling, ethical considerations become paramount. Bias in training data can lead to biased AI models, perpetuating existing societal inequalities. Remotasks will need to implement robust mechanisms to detect and mitigate bias, ensuring fairness and inclusivity in the data it provides. This could involve careful selection of annotators from diverse backgrounds and the development of algorithms that actively identify and correct for bias in the annotation process. Transparency and accountability are also key, with clear guidelines and mechanisms for addressing potential ethical concerns.
Impacts of Increased Automation on Data Labeling Jobs
The increasing automation of data labeling tasks will undoubtedly impact the roles of human annotators. While some tasks may become automated, new roles will emerge requiring specialized skills in data management, quality control, and AI-assisted annotation. For instance, annotators may focus on more complex and nuanced tasks that require human judgment and expertise, while AI handles the more repetitive aspects of the process. Remotasks will need to invest in training and upskilling its workforce to ensure a smooth transition and to create new opportunities for its annotators. This could involve offering specialized training programs in AI-related fields, fostering a culture of continuous learning, and providing career advancement opportunities. A successful transition requires a proactive approach that balances the benefits of automation with the needs of the human workforce.
Case Studies of Successful Projects
Remotasks has been instrumental in powering cutting-edge AI projects across diverse sectors. The success of these ventures hinges on the high-quality data labeling provided by its global network of expert annotators, guided and supported by AI specialists. Let’s delve into a few compelling examples.
Project Name | Data Annotated | Challenges Faced | Results Achieved |
---|---|---|---|
Autonomous Vehicle Perception | Images and LiDAR data for object detection (pedestrians, vehicles, traffic signs) and scene understanding. | Ensuring consistent and accurate annotation across a vast dataset; managing the complexity of different sensor modalities; dealing with edge cases and ambiguous situations in real-world driving scenarios. | Improved accuracy of object detection and scene understanding in autonomous driving systems, leading to safer and more reliable vehicles. The resulting AI model demonstrated a significant reduction in false positives and improved recall, directly translating to enhanced performance in real-world testing. |
Medical Image Analysis | CT scans and MRI images for lesion detection and classification in oncology. | High level of accuracy required due to the critical nature of medical diagnoses; managing inter-annotator variability and ensuring consistency across different radiologists’ interpretations; dealing with the inherent noise and variability in medical images. | Improved diagnostic accuracy in detecting cancerous lesions, enabling earlier and more effective treatment interventions. The AI model showed a significant improvement in sensitivity and specificity compared to traditional methods, leading to faster and more accurate diagnoses. The high-quality data labeling directly contributed to the model’s ability to distinguish subtle variations in tissue density and texture, crucial for accurate lesion identification. |
Satellite Imagery Analysis | High-resolution satellite images for land cover classification (e.g., identifying deforestation, urban sprawl, agricultural land). | Dealing with the vast volume of data; ensuring consistent annotation across different geographical regions and varying image qualities; managing the complexities of cloud cover and shadows. | Improved accuracy in monitoring environmental changes and urban development. The AI model enabled more efficient and accurate analysis of large-scale satellite imagery, providing valuable insights for environmental monitoring, urban planning, and disaster response. The impact of high-quality data labeling was evident in the model’s ability to accurately classify different land cover types even in challenging conditions, such as areas with partial cloud cover or varying lighting conditions. |
AI Expert Involvement in Successful Projects, Remotasks ai expert data labor
AI experts played a crucial role in all three projects, guiding the annotation process, defining annotation guidelines, and ensuring data quality. Their expertise was vital in addressing complex challenges, defining clear annotation protocols, and optimizing the workflow for maximum efficiency and accuracy. They provided crucial feedback to the annotators, resolved discrepancies, and ensured the final dataset met the rigorous standards required for training high-performing AI models. Their contribution extended beyond simple quality control; they actively shaped the project’s success by ensuring the data accurately reflected the nuances and complexities of the task.
Impact of High-Quality Data Labeling on Final AI Models
The impact of high-quality data labeling on the final AI models was transformative. In each case, the meticulous annotation directly translated to improved model accuracy, robustness, and generalizability. Imagine trying to teach a child to identify a cat: showing them only blurry, poorly lit pictures will result in a child who can’t reliably identify a cat in various lighting conditions. Similarly, using low-quality data to train an AI model leads to poor performance and unreliable predictions. Conversely, providing the AI model with precise, consistently labeled data enables it to learn effectively and generalize its knowledge to new, unseen data, resulting in superior performance and real-world applicability. The difference between a model trained on meticulously labeled data and one trained on poorly labeled data can be the difference between a life-saving medical diagnosis and a missed opportunity, or between a self-driving car that safely navigates traffic and one that causes an accident. The investment in high-quality data labeling pays off exponentially in the performance and reliability of the final AI model.
Last Recap
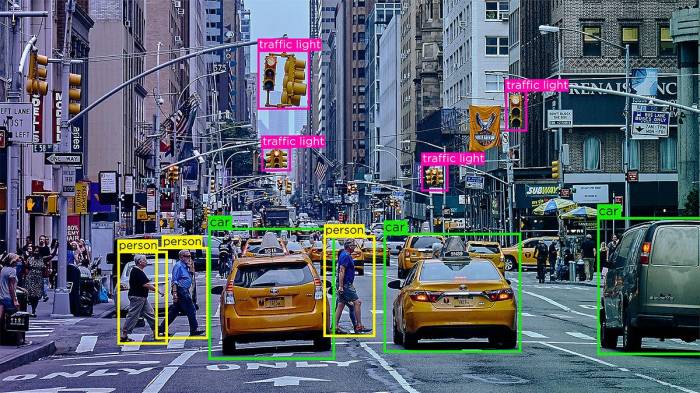
Source: medium.com
The world of Remotasks AI expert data labor is a dynamic intersection of human ingenuity and cutting-edge technology. While challenges exist, the rewards – from shaping the future of AI to contributing to groundbreaking innovations – are immense. As AI continues to evolve, the demand for skilled data annotators will only increase, highlighting the crucial role these experts play in building a more intelligent and interconnected world. The future of AI isn’t just coded; it’s meticulously labeled, one data point at a time, by the unsung heroes of Remotasks.