Use of ai is seeping into academic journals and its proving difficult to detect – AI’s use is seeping into academic journals and its proving difficult to detect. Think ghostwritten papers, subtly altered sentences, entire sections crafted by algorithms – the academic world is facing a silent revolution. This isn’t just about plagiarism in the traditional sense; it’s a new level of academic dishonesty, far more sophisticated and harder to pinpoint. The implications are huge, impacting everything from research integrity to the very fabric of academic trust.
This insidious infiltration poses a significant threat to the academic ecosystem. Journal editors, already burdened with a heavy workload, now face the daunting task of identifying AI-generated content amidst a sea of legitimate submissions. Existing plagiarism detection tools often fall short, highlighting the urgent need for innovative detection methods and robust ethical guidelines.
The Prevalence of AI-Generated Text in Academic Journals: Use Of Ai Is Seeping Into Academic Journals And Its Proving Difficult To Detect
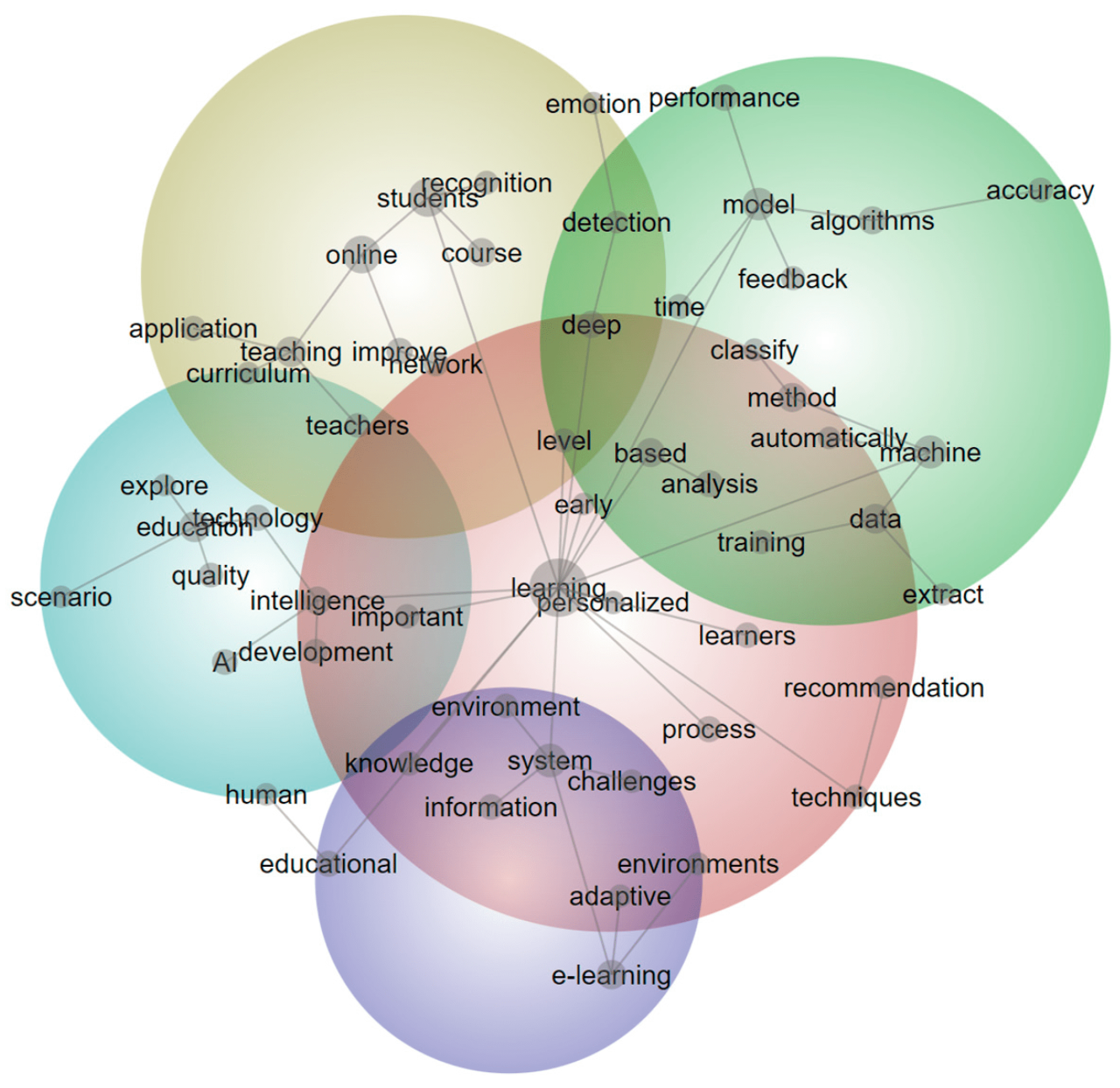
Source: mdpi-res.com
The quiet infiltration of AI into academic writing is causing a significant ripple effect across the scholarly landscape. While the technology offers potential benefits like enhanced writing assistance, the ease with which AI can generate seemingly original text raises serious concerns about academic integrity and the validity of published research. The challenge now lies in detecting this subtle form of plagiarism, a task that is proving increasingly difficult for journal editors and reviewers.
The current landscape reveals a growing reliance on AI tools, particularly among researchers facing tight deadlines or struggling with complex writing tasks. The anonymity offered by these tools makes them attractive to those seeking to cut corners, even unintentionally. This trend is impacting various disciplines, blurring the lines between human-authored and AI-assisted work.
Methods of AI-Generated Academic Content
Several AI writing tools are readily available, offering varying degrees of sophistication. These range from simple grammar and style checkers to advanced language models capable of generating entire essays or research papers. Common methods involve feeding the AI with s, abstracts, or existing literature on a specific topic, prompting the AI to generate text based on that input. Some researchers might use AI to overcome writer’s block, refine their arguments, or even create drafts that are subsequently edited and polished. Others, however, might submit AI-generated content with minimal or no alterations.
Examples of AI-Generated Content in Academic Papers
AI-generated text can infiltrate various types of academic papers. It could be present in literature reviews, where AI might synthesize information from multiple sources, potentially omitting crucial nuances or critical analyses. Similarly, it could be found in discussion sections, where AI might generate summaries or interpretations of findings without deep engagement with the underlying data. Even seemingly original research papers might contain AI-generated sections, such as introductions or conclusions, masking the actual extent of AI involvement. The potential for this type of undetected AI-generated content exists across disciplines, from the humanities to STEM fields.
Challenges in Detecting AI-Generated Content, Use of ai is seeping into academic journals and its proving difficult to detect
Detecting AI-generated text presents unique challenges for journal editors. Traditional plagiarism detection software focuses on identifying verbatim copying from existing sources. However, AI-generated text is often paraphrased and syntactically varied, making it difficult for these tools to flag. Furthermore, the constant evolution of AI models makes it a moving target for detection methods. The subtle stylistic inconsistencies or unnatural phrasing that might signal AI authorship can be easily missed, especially in the context of a lengthy research paper.
Comparison of Plagiarism Detection Methods
Method | Strengths | Weaknesses | Applicability to AI-generated text |
---|---|---|---|
Traditional Plagiarism Detection Software | Identifies verbatim copying; widely available and relatively inexpensive. | Ineffective against paraphrased content; struggles with AI-generated text. | Low; detects only direct copying, not AI-generated paraphrases. |
AI Detection Software (e.g., GPTZero, Originality.ai) | Specifically designed to identify AI-generated text; can detect paraphrased content. | Accuracy varies depending on the AI model used; false positives are possible. | High; provides a more reliable detection rate than traditional methods. |
Manual Review by Experts | Can identify subtle stylistic inconsistencies and unnatural phrasing; considers context and argumentation. | Time-consuming and expensive; requires expertise in the specific field. | Moderate to High; effective but resource-intensive. |
Techniques for Detecting AI-Generated Academic Content
The rise of sophisticated AI writing tools presents a significant challenge to academic integrity. While plagiarism detection software has long been a staple in ensuring originality, its effectiveness against AI-generated text is severely limited, demanding the development of more nuanced detection methods. This section explores current techniques and proposes a novel approach to identify AI-written academic content.
Existing plagiarism detection software primarily relies on comparing submitted text against a vast database of existing publications. This approach is effective in identifying direct copying but falls short when dealing with AI-generated text, which often paraphrases and restructures information in ways that evade traditional plagiarism checkers. These tools struggle because AI-generated content is often grammatically correct and semantically coherent, lacking the telltale signs of typical plagiarism, such as verbatim copying or poorly integrated paraphrases. Furthermore, the rapid evolution of AI models means that existing databases quickly become outdated, leaving a significant gap in detection capabilities.
Limitations of Existing Plagiarism Detection Software
Current plagiarism detection software, while useful for identifying direct copying and blatant plagiarism, struggles with the subtle nuances of AI-generated text. They often miss instances of paraphrasing and rewording, which are common techniques used by AI to avoid detection. The sheer volume of text generated by AI models also overwhelms the capacity of these systems to effectively compare and contrast. For example, a tool might flag a sentence as potentially plagiarized because it shares a few s with an existing publication, even if the overall meaning and context are different. This leads to false positives and hinders the accuracy of the detection process. Moreover, the rapid advancement of AI language models necessitates continuous updates to the software’s databases, a challenge that many existing solutions struggle to keep pace with.
Novel Approaches to Detecting AI-Generated Text
Beyond the limitations of traditional plagiarism checkers, novel approaches leverage stylistic analysis and pattern recognition to identify AI-generated content. These techniques analyze the writing style at a granular level, looking for patterns and inconsistencies that are characteristic of AI-generated text. For example, AI models often exhibit a predictable sentence structure or overuse certain phrases and vocabulary, creating a stylistic fingerprint. Analyzing sentence length variation, the frequency of specific word combinations, and the use of complex grammatical structures can reveal subtle differences between human-written and AI-generated text. Furthermore, sophisticated algorithms can identify patterns in the way AI models process and organize information, revealing inconsistencies or irregularities not found in human writing. These methods can be combined to build a robust detection system.
Comparison of Algorithms for Identifying AI-Written Content
Several algorithms are being developed to specifically target AI-generated text. Some focus on statistical analysis of word frequencies and sentence structures, while others employ machine learning models trained on large datasets of both human-written and AI-generated text. These models learn to identify subtle stylistic differences and patterns that distinguish the two. For instance, some algorithms analyze the perplexity of the text – a measure of how surprising or unexpected the word choices are. High perplexity might indicate human writing, while low perplexity could suggest AI generation, although this isn’t foolproof. Other algorithms might analyze the coherence and fluency of the text using natural language processing techniques, looking for inconsistencies or unnatural phrasing that betray the AI’s underlying logic. A direct comparison is difficult because the effectiveness of each algorithm depends heavily on the specific AI model used to generate the text and the dataset used to train the detection algorithm.
Hypothetical Algorithm for Detecting AI-Generated Text
A hypothetical algorithm could combine several approaches for improved accuracy. It would begin with a pre-processing stage to clean the text and remove irrelevant information. Next, it would analyze the text’s stylistic features, including sentence length variation, word frequency, and the use of complex grammatical structures. These features would be compared against a database of known human-written and AI-generated text. The algorithm would then employ a machine learning model, specifically a Random Forest classifier, trained on this database to classify the input text as either human-written or AI-generated. The Random Forest approach is chosen for its robustness and ability to handle high-dimensional data. The output would be a probability score indicating the likelihood that the text was generated by AI. The algorithm’s limitations would include its dependence on the quality and size of the training dataset and the potential for AI models to adapt and evade detection over time. False positives and negatives would still be possible, highlighting the need for ongoing refinement and adaptation.
Ethical and Academic Implications of AI-Generated Content
The rise of AI writing tools presents a complex ethical dilemma for academia. While offering potential benefits like increased efficiency, these tools also introduce significant risks to the integrity of research and the fairness of academic processes. The ease with which AI can generate seemingly original text raises serious concerns about plagiarism, authorship, and the very definition of scholarly contribution. Understanding these implications is crucial for navigating this rapidly evolving landscape.
The potential for misuse of AI in academic writing is substantial. Students might leverage AI to complete assignments without genuine understanding, submitting work that lacks intellectual depth and originality. Researchers could similarly use AI to generate sections of papers or even entire manuscripts, potentially misrepresenting their contributions and undermining the peer-review process. This could lead to the publication of inaccurate or misleading research, eroding public trust in academic scholarship. Imagine, for example, a researcher using AI to fabricate data supporting a particular hypothesis, leading to potentially harmful conclusions being disseminated widely. Or consider a student submitting an AI-generated essay as their own work, gaining an unfair academic advantage.
Impact of AI-Generated Content on the Integrity of Academic Research
AI-generated content directly threatens the core principles of academic research: originality, honesty, and rigor. The ability of AI to mimic various writing styles makes detecting plagiarism significantly more challenging, blurring the lines between legitimate paraphrasing and outright fabrication. Furthermore, the use of AI in data analysis or interpretation can lead to biased or inaccurate results if not carefully scrutinized. The very process of research, involving critical thinking, intellectual exploration, and the development of original arguments, is bypassed when AI is used to generate content. This undermines the intellectual growth and development that are essential aspects of the academic process. For instance, a researcher using AI to write a literature review might miss crucial nuances or alternative interpretations, resulting in a flawed understanding of the subject matter.
Exacerbation of Existing Inequalities in Academic Publishing
The accessibility of AI writing tools could exacerbate existing inequalities in academic publishing. Researchers with greater resources might have more opportunities to leverage AI’s capabilities, potentially gaining an unfair advantage over those with limited access to such technologies. This could further marginalize underrepresented groups in academia and perpetuate existing biases within the research landscape. For example, researchers at well-funded institutions might use AI to accelerate their publication rates, leaving behind researchers at less well-resourced institutions who lack access to these tools. This unequal access could lead to a skewed representation of research perspectives and a further concentration of power within academia.
Ethical Guidelines for Researchers Using AI in Their Work
The ethical use of AI in academic research requires a clear set of guidelines. Researchers must prioritize transparency, accountability, and intellectual honesty. The following principles should guide the responsible integration of AI into the academic process:
- Transparency: Clearly disclose the use of AI tools in all stages of research, including data collection, analysis, and writing.
- Attribution: Properly attribute any AI-generated content, avoiding misrepresentation of authorship.
- Critical Evaluation: Thoroughly review and critically evaluate any output generated by AI tools, ensuring accuracy and avoiding reliance on flawed or biased results.
- Intellectual Honesty: Maintain the highest standards of intellectual honesty, avoiding plagiarism and ensuring that all work reflects original thought and contribution.
- Responsible Use: Utilize AI tools responsibly, recognizing their limitations and potential biases, and avoiding their misuse for unethical purposes.
The Future of AI and Academic Integrity
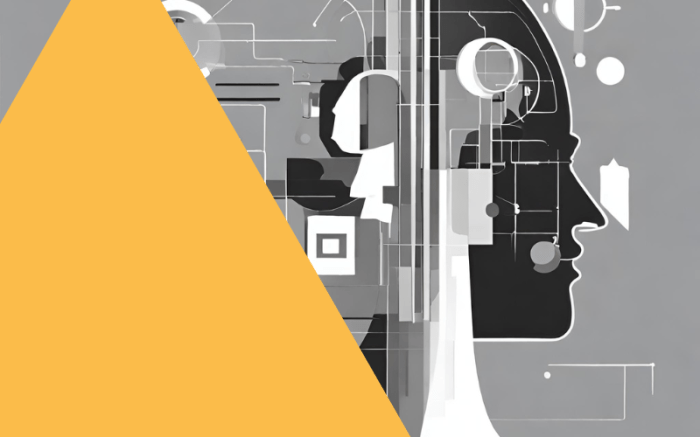
Source: broneager.com
The rise of AI writing tools has thrown a wrench into the gears of academic integrity, forcing a rapid evolution in how we approach plagiarism and authenticity. The future hinges on a technological arms race between AI generators and detection systems, coupled with a significant shift in pedagogical approaches and institutional policies. This isn’t just about catching cheaters; it’s about redefining what constitutes original scholarship in the age of artificial intelligence.
AI detection technology will likely become significantly more sophisticated in the coming years. We can expect advancements in techniques that analyze stylistic nuances, contextual understanding, and even the subtle patterns of thought embedded within text. Think beyond simple matching; imagine AI detectors that analyze the probability distribution of sentence structures, the originality of arguments, and even the emotional tone of the writing – all indicators that could reveal AI authorship. For example, current tools often struggle with nuanced academic prose, but future iterations might incorporate machine learning models trained on vast datasets of peer-reviewed articles, enabling more accurate identification of AI-generated content.
AI’s Dual Role in Academic Integrity
AI poses a significant threat to academic integrity by enabling the easy creation of high-quality, seemingly original essays, research papers, and even code. Students could easily bypass traditional plagiarism detection methods, submitting work that’s technically unique but fundamentally dishonest. However, AI also offers potential benefits. AI-powered plagiarism detection tools, as previously discussed, are becoming increasingly effective. Furthermore, AI can assist educators in identifying patterns of academic misconduct, flagging suspicious submissions for further review. This could involve AI analyzing writing styles across multiple assignments from a single student to pinpoint inconsistencies that might indicate AI usage. The key is to harness the power of AI for good while mitigating its potential for misuse.
Adapting Institutional Policies and Procedures
Universities and other educational institutions need to proactively adapt their policies and procedures to address the challenges posed by AI-generated content. This involves more than simply updating plagiarism policies; it requires a fundamental rethinking of assessment methods. For example, institutions could shift towards more emphasis on in-class assessments, oral presentations, and project-based learning, where AI assistance is less effective. Furthermore, incorporating AI literacy into the curriculum is crucial, teaching students how to use AI responsibly and ethically while recognizing its limitations. Clear guidelines regarding the acceptable use of AI in academic work need to be established and communicated effectively to students. This might include mandating the declaration of AI tool usage for specific tasks, fostering a culture of transparency and accountability. Consider the University of Cambridge’s approach – they are actively exploring ways to integrate AI detection into their assessment processes, while also emphasizing the importance of responsible AI usage.
A Future Shaped by AI in Academia
Imagine a future where AI plays a central role in both creating and detecting academic dishonesty. AI writing tools become even more sophisticated, capable of producing highly nuanced and contextually relevant academic work, while simultaneously, AI detection systems become equally adept at identifying AI-generated text. This could lead to a constant cycle of technological innovation, pushing the boundaries of what constitutes “original” work. The societal impact could be profound. The value of human intellect and critical thinking skills might be further emphasized, as the ability to synthesize information creatively and critically would become even more valuable than the ability to simply generate text. The focus might shift from simply producing content to demonstrating genuine understanding and intellectual contribution. However, this scenario also carries risks: a potential widening of the academic achievement gap, with students from less privileged backgrounds lacking access to advanced AI tools or the digital literacy to navigate this evolving landscape. This necessitates equitable access to technology and educational resources, ensuring that all students have the opportunity to thrive in this AI-driven academic environment.
Case Studies of AI-Generated Academic Papers
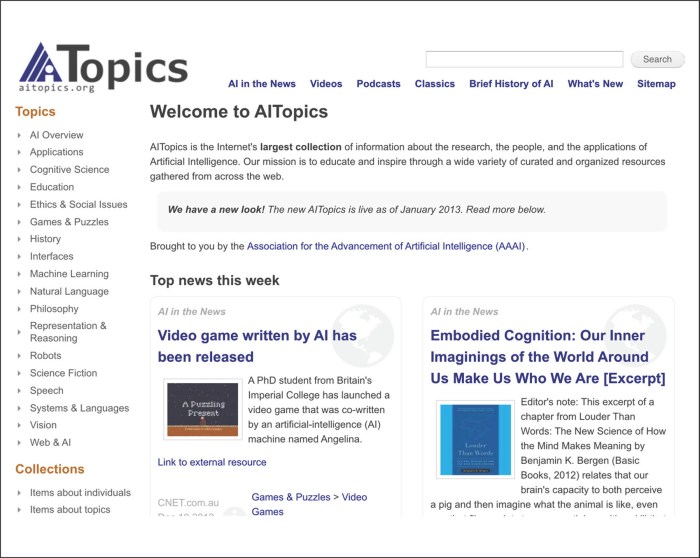
Source: wiley.com
The insidious creep of AI into academic publishing presents a complex challenge. Detecting AI-generated text isn’t always straightforward, leading to potential breaches of academic integrity and the distortion of scholarly discourse. Examining specific cases highlights the vulnerabilities and the need for robust detection methods.
The following case study explores a hypothetical scenario, illustrating the detection process and its consequences. It also explores the broader impact on a specific academic field.
A Hypothetical Case Study: The Fabricated Neuroscience Paper
Imagine a researcher, Dr. Anya Sharma, submits a paper on a groundbreaking new treatment for Alzheimer’s disease to a prestigious neuroscience journal. The paper, meticulously written and referencing numerous studies, proposes a novel approach with seemingly compelling data. Peer reviewers initially find the paper impressive, the methodology rigorous, and the results statistically significant. However, a closer examination, prompted by inconsistencies in the writing style compared to Dr. Sharma’s previous publications and a flagged similarity score from an AI detection tool, reveals a troubling truth: significant portions of the paper, including the results section, were generated using an advanced AI writing tool. The AI cleverly synthesized information from existing literature, creating a convincing but ultimately fabricated study. Upon investigation, Dr. Sharma admits to using AI to overcome writer’s block, believing the AI-generated text simply needed minor editing. The consequences are severe: retraction of the paper, damage to Dr. Sharma’s reputation, potential disciplinary action from her university, and a significant erosion of trust in the journal’s peer-review process.
Impact on the Neuroscience Field
This hypothetical case, while fictional, reflects a real concern within neuroscience and other fields heavily reliant on empirical data. The publication of fraudulent AI-generated research could lead to wasted resources, misdirected research efforts, and, most critically, potentially harmful consequences for patients. For example, if clinicians based treatment protocols on the fabricated findings, it could delay the development of genuinely effective therapies and even cause harm. The long-term consequences include a decline in the credibility of published research, increased skepticism among the scientific community, and a potential chilling effect on genuine innovation. The need for rigorous verification and improved detection methods becomes paramount to safeguard the integrity of the field.
Visual Representation of AI-Generated Content Spread
Imagine a network graph. Each node represents a different academic discipline (e.g., medicine, engineering, humanities). The nodes are connected by lines, representing the flow of information and collaboration between disciplines. The thickness of each line corresponds to the volume of interdisciplinary research. Now, imagine that some nodes, initially small and sparsely connected, begin to grow rapidly. These nodes represent disciplines where AI-generated content is becoming increasingly prevalent. The lines connecting these rapidly growing nodes to other disciplines thicken, indicating the spread of AI-generated text across various fields. The visual emphasizes the potential for a rapid, cascading effect as AI-generated content permeates the academic landscape, highlighting the urgency of addressing this issue.
Ending Remarks
The rise of AI in academic writing presents a complex challenge, forcing a re-evaluation of traditional methods of ensuring academic integrity. While the technology offers potential benefits, its misuse threatens the very foundations of scholarship. The future requires a multi-pronged approach: developing advanced detection technologies, establishing clear ethical guidelines, and fostering a culture of academic honesty. Ignoring this challenge risks eroding the credibility and trustworthiness of academic research, leaving us with a system vulnerable to manipulation and deceit. The race to detect and deter AI-generated academic dishonesty is far from over; it’s only just begun.